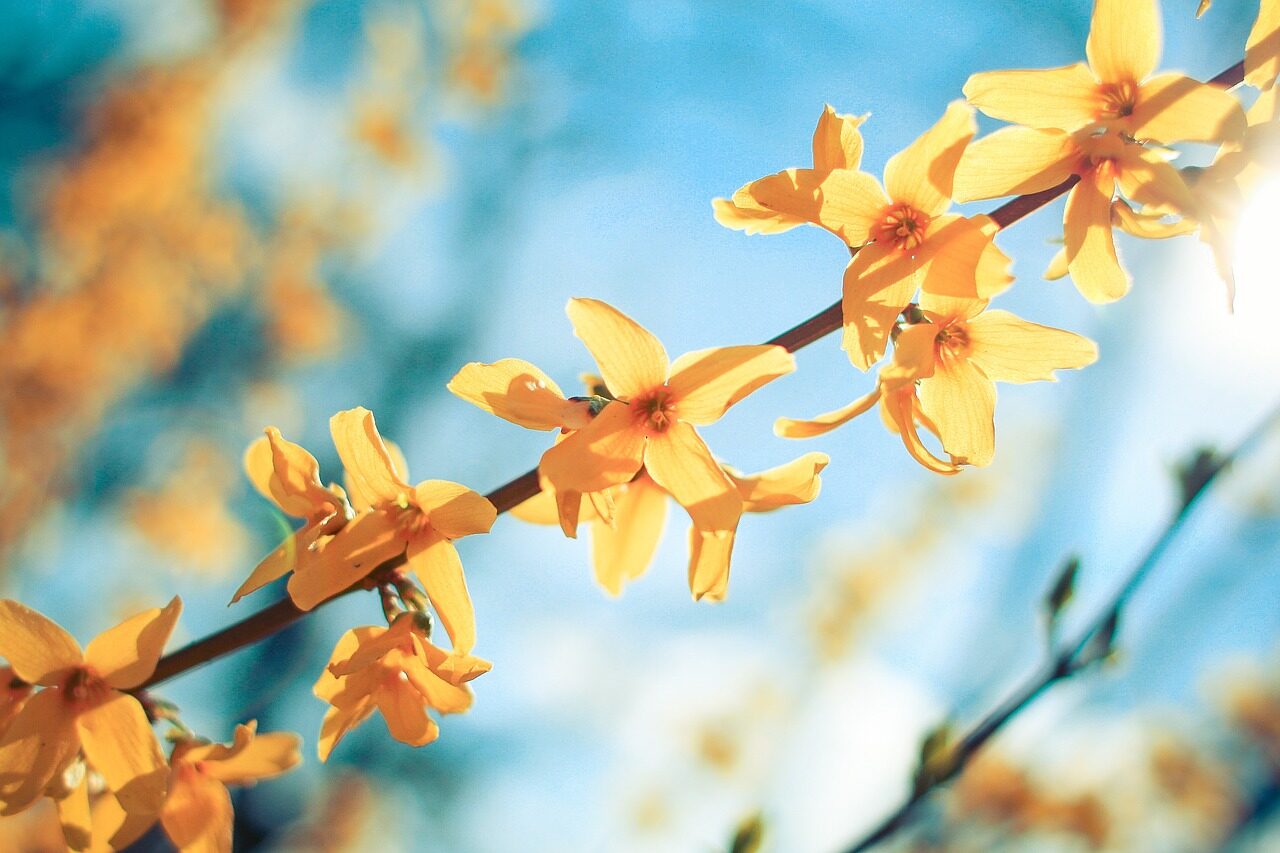
本文最初发布于 Towards Data Science。
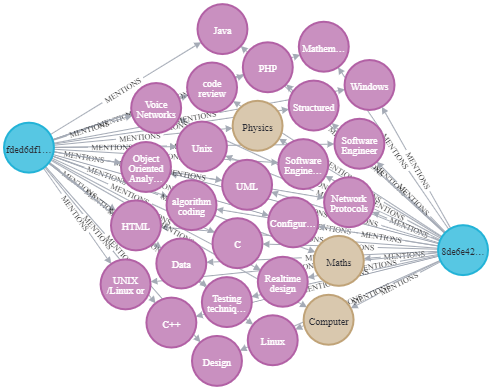
简介
在这篇文章中,我将展示如何使用经过优化的、基于转换器的命名实体识别(NER)以及 spaCy 的关系提取模型,基于职位描述创建一个知识图谱。这里介绍的方法可以应用于其他任何领域,如生物医学、金融、医疗保健等。
以下是我们要采取的步骤:
在 Google Colab 中加载优化后的转换器 NER 和 spaCy 关系提取模型;
创建一个 Neo4j Sandbox,并添加实体和关系;
查询图,找出与目标简历匹配度最高的职位,找出三个最受欢迎的技能和共现率最高的技能。
要了解关于如何使用 UBIAI 生成训练数据以及优化 NER 和关系提取模型的更多信息,请查看以下文章。
职位描述数据集可以从Kaggle获取。
在本文结束的时候,我们就可以创建出如下所示的知识图谱。
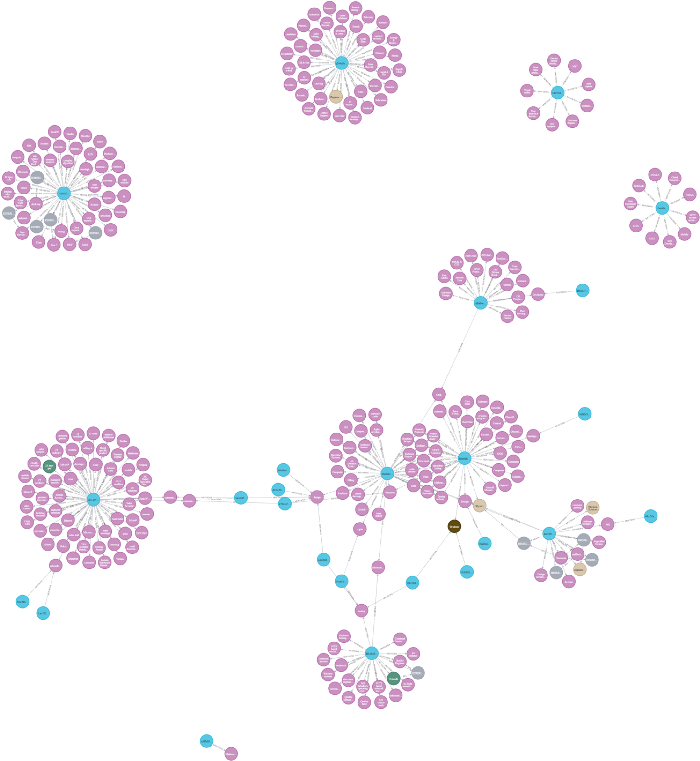
命名实体和关系提取
首先,我们加载 NER 和关系模型的依赖关系,以及之前优化过的 NER 模型本身,以提取技能、学历、专业和工作年限:
!pip install -U pip setuptools wheel
!python -m spaCy project clone tutorials/rel_component
!pip install -U spaCy-nightly --pre
!!pip install -U spaCy transformers
import spaCy
#安装完依赖项后重启运行时
nlp = spaCy.load("[PATH_TO_THE_MODEL]/model-best")
加载我们想从中提取实体和关系的职位数据集:
import pandas as pd
def get_all_documents():
df = pd.read_csv("/content/drive/MyDrive/job_DB1_1_29.csv",sep='"',hea
der=None)
documents = []
for index,row in df.iterrows():
documents.append(str(row[0]))
return documents
documents = get_all_documents()
documents = documents[:]
从职位数据集中提取实体:
import hashlib
def extract_ents(documents,nlp):
docs = list()
for doc in nlp.pipe(documents, disable=["tagger", "parser"]):
dictionary=dict.fromkeys(["text", "annotations"])
dictionary["text"]= str(doc)
dictionary['text_sha256'] = hashlib.sha256(dictionary["text"].encode('utf-8')).hexdigest()
annotations=[]
for e in doc.ents:
ent_id = hashlib.sha256(str(e.text).encode('utf-8')).hexdigest()
ent = {"start":e.start_char,"end":e.end_char, "label":e.label_,"label_upper":e.label_.upper(),"text":e.text,"id":ent_id}
if e.label_ == "EXPERIENCE":
ent["years"] = int(e.text[0])
annotations.append(ent)
dictionary["annotations"] = annotations
docs.append(dictionary)
#print(annotations)
return docs
parsed_ents = extract_ents(documents,nlp)
在将实体提供给关系提取模型之前,我们可以看下提取出的部分实体:
[('stock market analysis', 'SKILLS'),
('private investor', 'SKILLS'),
('C++', 'SKILLS'),
('Investment Software', 'SKILLS'),
('MS Windows', 'SKILLS'),
('web development', 'SKILLS'),
('Computer Science', 'DIPLOMA_MAJOR'),
('AI', 'SKILLS'),
('software development', 'SKILLS'),
('coding', 'SKILLS'),
('C', 'SKILLS'),
('C++', 'SKILLS'),
('Visual Studio', 'SKILLS'),
('2 years', 'EXPERIENCE'),
('C/C++ development', 'SKILLS'),
('data compression', 'SKILLS'),
('financial markets', 'SKILLS'),
('financial calculation', 'SKILLS'),
('GUI design', 'SKILLS'),
('Windows development', 'SKILLS'),
('MFC', 'SKILLS'),
('Win', 'SKILLS'),
('HTTP', 'SKILLS'),
('TCP/IP', 'SKILLS'),
('sockets', 'SKILLS'),
('network programming', 'SKILLS'),
('System administration', 'SKILLS')]
我们现在准备好预测关系了;首先加载关系提取模型,务必将目录改为 rel_component/scripts 以便可以访问关系模型的所有必要脚本。
cd rel_component/
import random
import typer
from pathlib import Path
import spaCy
from spaCy.tokens import DocBin, Doc
from spaCy.training.example import Example
#使factory生效
from rel_pipe import make_relation_extractor, score_relations
#使config生效
from rel_model import create_relation_model, create_classification_layer, create_instances, create_tensors
#安装完依赖项后重启运行时
nlp2 = spaCy.load("/content/drive/MyDrive/training_rel_roberta/model-best")
def extract_relations(documents,nlp,nlp2):
predicted_rels = list()
for doc in nlp.pipe(documents, disable=["tagger", "parser"]):
source_hash = hashlib.sha256(doc.text.encode('utf-8')).hexdigest()
for name, proc in nlp2.pipeline:
doc = proc(doc)
for value, rel_dict in doc._.rel.items():
for e in doc.ents:
for b in doc.ents:
if e.start == value[0] and b.start == value[1]:
max_key = max(rel_dict, key=rel_dict. get)
#print(max_key)
e_id = hashlib.sha256(str(e).encode('utf-8')).hexdigest()
b_id = hashlib.sha256(str(b).encode('utf-8')).hexdigest()
if rel_dict[max_key] >=0.9 :
#print(f" entities: {e.text, b.text} --> predicted relation: {rel_dict}")
predicted_rels.append({'head': e_id, 'tail': b_id, 'type':max_key, 'source': source_hash})
return predicted_rels
predicted_rels = extract_relations(documents,nlp,nlp2)
Predicted relations:
entities: ('5+ years', 'software engineering') --> predicted relation: {'DEGREE_IN': 9.5471655e-08, 'EXPERIENCE_IN': 0.9967771}
entities: ('5+ years', 'technical management') --> predicted relation: {'DEGREE_IN': 1.1285037e-07, 'EXPERIENCE_IN': 0.9961034} entities: ('5+ years', 'designing') --> predicted relation:{'DEGREE_IN': 1.3603304e-08, 'EXPERIENCE_IN': 0.9989103}
entities: ('4+ years', 'performance management') --> predicted relation: {'DEGREE_IN': 6.748373e-08, 'EXPERIENCE_IN': 0.92884386}
Neo4J
现在,我们可以加载职位数据集,并将数据提取到 Neo4j 数据库中了。
首先,我们创建一个空的Neo4j Sandbox,并添加连接信息,如下所示:
documents = get_all_documents()
documents = documents[:]
parsed_ents = extract_ents(documents,nlp)
predicted_rels = extract_relations(documents,nlp,nlp2)
#neo4j的基础查询功能
from neo4j import GraphDatabase
import pandas as pd
host = 'bolt://[your_host_address]'
user = 'neo4j'
password = '[your_password]'
driver = GraphDatabase.driver(host,auth=(user, password))
def neo4j_query(query, params=None):
with driver.session() as session:
result = session.run(query, params)
return pd.DataFrame([r.values() for r in result], columns=result.keys())
接下来,我们将文档、实体和关系添加到知识图谱中。注意,我们需要从实体 EXPERIENCE 的 name 中提取出整数年限,并将其作为一个属性存储起来。
#清空当前的Neo4j Sandbox db (删除所有东西)
neo4j_query("""
MATCH (n) DETACH DELETE n;
""")
#创建第一个主节点
neo4j_query("""
MERGE (l:LaborMarket {name:"Labor Market"})
RETURN l
""")
#向KG中添加实体:技能、经验、学历、专业
neo4j_query("""
MATCH (l:LaborMarket)
UNWIND $data as row
MERGE (o:Offer{id:row.text_sha256})
SET o.text = row.text
MERGE (l)-[:HAS_OFFER]->(o)
WITH o, row.annotations as entities
UNWIND entities as entity
MERGE (e:Entity {id:entity.id})
ON CREATE SET
e.name = entity.text,
e.label = entity.label_upper
MERGE (o)-[m:MENTIONS]->(e)
ON CREATE SET m.count = 1
ON MATCH SET m.count = m.count + 1
WITH e as e
CALL apoc.create.addLabels( id(e), [ e.label ] )
YIELD node
REMOVE node.label
RETURN node
""", {'data': parsed_ents})
#为实体EXPERIENCE添加属性'name'
res = neo4j_query("""
MATCH (e:EXPERIENCE)
RETURN e.id as id, e.name as name
""")
#从EXPERIENCE name中提取工作年限,并保存在属性years中
import re
def get_years(name):
return re.findall(r"\d+",name)[0]
res["years"] = res.name.map(lambda name: get_years(name))
data = res.to_dict('records')
#为实体EXPERIENCE添加属性'years'
neo4j_query("""
UNWIND $data as row
MATCH (e:EXPERIENCE {id:row.id})
SET e.years = row.years
RETURN e.name as name, e.years as years
""",{"data":data})
#将关系添加到KG
neo4j_query("""
UNWIND $data as row
MATCH (source:Entity {id: row.head})
MATCH (target:Entity {id: row.tail})
MATCH (offer:Offer {id: row.source})
MERGE (source)-[:REL]->(r:Relation {type: row.type})-[:REL]->(target)
MERGE (offer)-[:MENTIONS]->(r)
""", {'data': predicted_rels})
现在开始进入有趣的部分了。我们可以启动知识图谱并运行查询了。让我们运行一个查询,找出与目标简历最匹配的职位:
#在表中显示最佳匹配项
other_id = "8de6e42ddfbc2a8bd7008d93516c57e50fa815e64e387eb2fc7a27000ae904b6"
query = """
MATCH (o1:Offer {id:$id})-[m1:MENTIONS]->(s:Entity)<- [m2:MENTIONS]-(o2:Offer)
RETURN DISTINCT o1.id as Source,o2.id as Proposed_Offer, count(*) as freq, collect(s.name) as common_terms
ORDER BY freq
DESC LIMIT $limit
"""
res = neo4j_query(query,{"id":other_id,"limit":3})
res
#在neo4j浏览器中,使用该查询显示最佳匹配项的图
"""MATCH (o1:Offer {id:"8de6e42ddfbc2a8bd7008d93516c57e50fa815e64e387eb2fc7a27000ae904b6"})-[m1:MENTIONS]->(s:Entity)<- [m2:MENTIONS]-(o2:Offer)
WITH o1,s,o2, count(*) as freq
MATCH (o1)--(s)
RETURN collect(o2)[0], o1,s, max(freq)"""
以表格形式显示的结果中的公共实体:
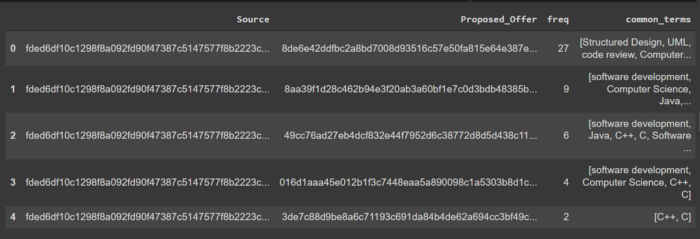
以可视化形式显示的图:
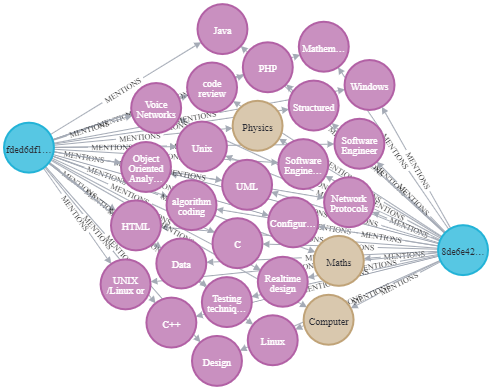
虽然这个数据集只有 29 个职位描述,但这里介绍的方法可以应用于有成千上万个职位的大规模数据集。只需几行代码,我们立马就可以提取出与目标简历匹配度最高的工作。
下面,让我们找出最需要的技能:
query = """
MATCH (s:SKILLS)<-[:MENTIONS]-(o:Offer)
RETURN s.name as skill, count(o) as freq
ORDER BY freq DESC
LIMIT 10
"""
res = neo4j_query(query)
res
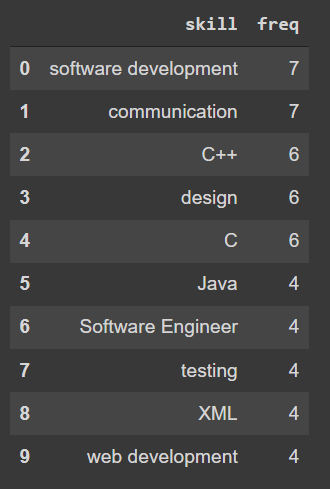
以及需要最高工作年限的技能:
query = """
MATCH (s:SKILLS)--(r:Relation)--(e:EXPERIENCE) where r.type = "EXPERIENCE_IN"
return s.name as skill,e.years as years
ORDER BY years DESC
LIMIT 10
"""
res = neo4j_query(query)
res
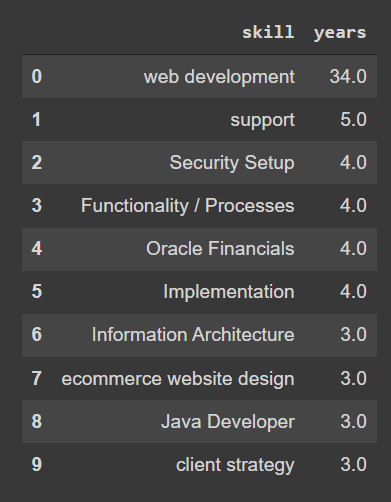
Web 开发和技术支持需要的工作年限最高,然后是安全设置。
最后,让我们查下共现率最高的技能对:
neo4j_query("""
MATCH (s1:SKILLS)<-[:MENTIONS]-(:Offer)-[:MENTIONS]->(s2:SKILLS)
WHERE id(s1) < id(s2)
RETURN s1.name as skill1, s2.name as skill2, count(*) as cooccurrence
ORDER BY cooccurrence
DESC LIMIT 5
""")
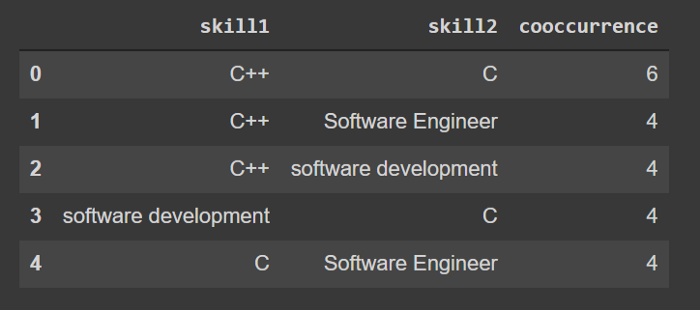
小结
在这篇文章中,我们描述了如何利用基于转换器的 NER 和 spaCy 的关系提取模型,用 Neo4j 创建知识图谱。除了信息提取之外,图的拓扑结构还可以作为其他机器学习模型的输入。
将 NLP 与图数据库 Neo4j 相结合,可以加速许多领域的信息发现,相比之下,在医疗和生物医学领域的应用效果更为显著。
如果你有任何问题或希望为具体用例创建自定义模型,请给我们发邮件(admin@ubiai.tools),或是在 Twitter 上给我们留言(@UBIAI5)。
原文链接:How to Build a Knowledge Graph with Neo4J and Transformers
更多内容推荐
2. SparkSQL 的 DataFrame 创建方式
2023-09-08
06|调用模型:使用 OpenAI API 还是微调开源 Llama2/ChatGLM?
在 LangChain 中使用自己微调的模型是完全没问题的。
2023-09-18
2021 云智技术论坛 - 知识智能化专场
随着人工智能技术的进步,智能化成为产业转型升级的重要抓手。企业要实现智能化升级,需要从呈几何增长的多模态数据中挖掘和凝炼知识、赋能业务,支撑商业模式转型和应用创新,推动业务高质量发展和产业革新。
使用 Amazon Rekognition 和图形数据库来了解电影明星的社交网络
Amazon Rekognition 是一种让您能够向应用程序中轻松添加图像分析功能的 AWS 服务。
用机器学习分析韩国流行音乐 4 - 生产模型
本文是本教程的第四部分,我将使用 FLASK 将预测模型投入生产。
10|CLIP:让 AI 绘画模型乖乖听你的话
只有真正理解了CLIP,你才能知道为什么prompt可以控制AI绘画生成的内容。
2023-08-07
使用 AWS Step Functions 和 AWS Glue 编排基于 Amazon Redshift 的 ETL 工作流(二)
Step Functions 编排
用机器学习分析流行音乐(四):生产模型
模型部署就是将机器学习模型集成到现有的生产环境中,以便基于数据做出实际的业务决策。
Java 近期新闻:Payara 平台、JReleaser、Quarkus、Hibernate、Spring Cloud 和 Apache Beam
最近,Java社区相对比较平静,本期的新闻包括JDK 19、JDK 20、Spring Cloud 2020.0.6、Quarkus 2.10.1、Payara平台企业版5.40.0。
小米在知识表示学习的探索与实践
知识表示是知识获取与应用的基础,贯穿了小米知识图谱的构建与应用。
LinkedIn 是如何用图神经网络扩充会员知识图谱的?
在这篇文章中,我们介绍了 Entity-BERT,它是一种新型的图神经网络,可以从现有的会员知识图谱中推理出缺失的会员实体。
15|检索增强生成:通过 RAG 助力鲜花运营
RAG 类的任务,目前企业实际应用场景中的需求量相当大,也是 LangChain 所关注的一个重点内容。
2023-10-11
【sql 语句基础】——查 (select)(单表查询)
-- GROUP by用于对查询的结果分组统计 -- 如何显示每个部门的平均工资和最高工资 SELECT AVG(sal), MAX(sal) , deptno FROM emp GROUP BY deptno; -- 使用数学方法,对小数点进行处理 SELECT FORMAT(AVG(sal),2), MAX(sal) , deptno
2022-06-12
仅用不到 150 行代码,我开发出了一个搜索引擎
在这篇文章中,我们将探索全文搜索引擎的基本组件,并用它们来构建一个可以搜索数百万个文档、根据相关性对文档进行排名的搜索引擎
从 Excel 到 Python
世界上发展最快的编程语言(Python)和世界上使用最为广泛的软件(Excel)之间的紧密集成可以给很多行业带来巨大收益。
华为联合中科大提出知识图谱结合预训练模型新方法,节省 20% 计算成本
如何将知识图谱的信息嵌入到预训练语言模型中,已经成为当前的研究热点。
07|效果炸裂的 PPT 图片处理技巧
2023-09-03
02|用 LangChain 快速构建基于“易速鲜花”本地知识库的智能问答系统
直观感受一下 LangChain 作为一个基于大语言模型的应用开发框架,功能到底有多么强大吧!
2023-09-11
宣布为 Apache MXNet 推出 ONNX 支持
今天,AWS 宣布推出 ONNX-MXNet,它是一种用于将 Open Neural Network Exchange (ONNX) 深度学习模型导入到 Apache MXNet 的开源 Python 程序包。
txtai:基于 Transformer 的人工智能搜索引擎
这是一个基于人工智能的搜索引擎,可以在任何应用程序中实现基于自然语言理解的搜索。
推荐阅读
DDD 项目落地之充血模型实践 | 京东云技术团队
2023-08-07
21|部署一个鲜花网络电商的人脉工具(下)
2023-10-25
02|实战:如何使用 Jupyter Notebook?
2023-11-13
快递单信息抽取【三】-- 五条标注数据提高准确率,仅需五条标注样本,快速完成快递单信息任务
2022-10-26
25|模型工程(一):如何让你的训练数据无中生有?
2023-10-16
Student Management System
2022-10-25
手把手教学小型金融知识图谱构建:量化分析、图数据库 neo4j、图算法、关系预测、命名实体识别、Cypher Cheetsheet 详细教学等
2023-07-08
电子书
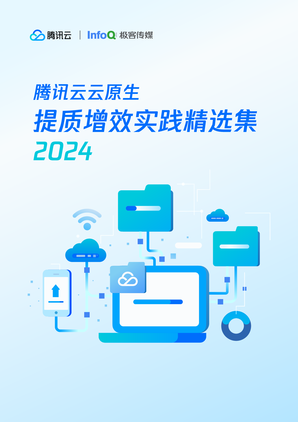
大厂实战PPT下载
换一换 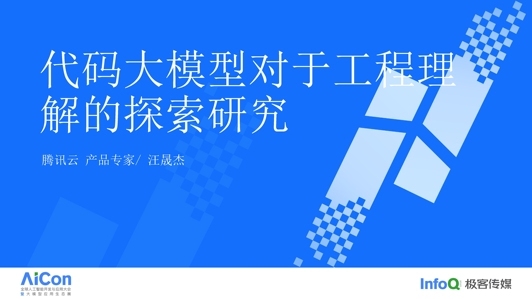
汪晟杰 | 腾讯云 产品专家
李龙彦 | OPPO 数智工程系统 / 安全与隐私部 / 网络技术专家
王城波 | 字节跳动 技术专家
评论