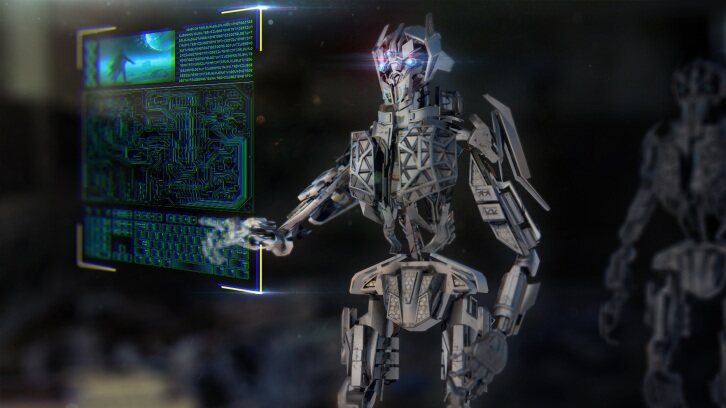
4 MNIST 深度卷积神经网络(CNN)
Softmax 性线回归网络中,输出 y 是输入 x 的线性组合,即 y = Wx+b,这是线性关系。在很多问题中其解法并非线性关系能完成的,在深度学习,能过能多层卷积神经网络组合非线性激活函数来模拟更复杂的非线性关系,效果往往比单一的线性关系更好。先看深度卷积神经网络(CNN,Convolutional Neural Network)构建的 MNIST 预测模型,再逐一介绍各网络层。
MNIST CNN Inference推理图。从输入到输出中间包含多个网络层:reshape、conv卷积、pool池化、fc全链接、dropout。自底向上输入原始图片数据x经过各层串行处理,得到各数字分类概率预测输出y。Inference的结果转给loss用作迭代训练,图中的

可以看出用的是 AdamOptimizer 优化器。
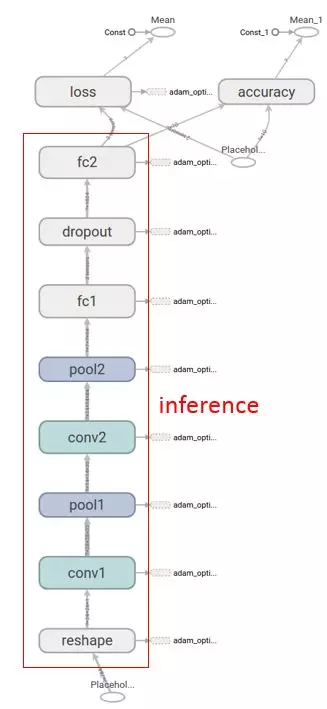
reshape 变形,对数据的逻辑结构进行改变,如二维变四维:[1, 784] => [1, 28, 28, 1],数据存储内容未发生改变。这里由于输入数据存储的手写图片是一维数据,转成[batch_size, height, width, channels]格式

conv2d 卷积, 卷积核(yellow)与Image元(green)素相乘,累加得到输出元素值(red)。Image的每个Channel(通道)都对应一个不同的卷积核,Channel内卷积核参数共享。所有输入channel与其kernel相乘累加多层得到输出的一个channel值。输出如有多个channel,则会重复多次,kernel也是不同的。所以会有input_channel_count * output_channel_count个卷积核。在卷积层中训练的是卷积核。

tf.nn.conv2d:
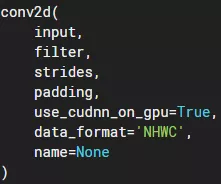
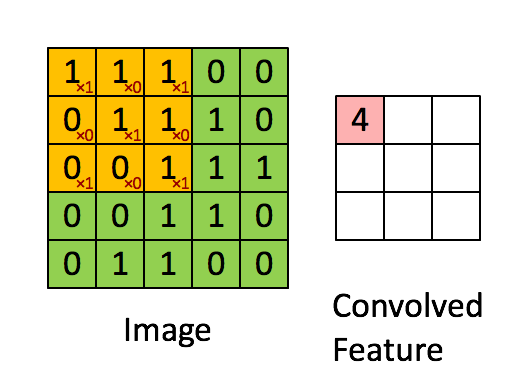
data_format: input和output数据的逻辑结构,NHWC : batch height width channel。NCHW: batch channel height width。常用的是NHWC格式;在一些输入数据中各通道数据分开存放,这种更适合NCHW。
input:输入,data_format=NHWC时,shape为batch, in_height, in_width, in_channels,Tensor。
filter:卷积核,shape为filter_height, filter_width, in_channels, out_channels,共有in_channels*out_channels个filter_height, filter_width的卷积核,输入输出channel越多,计算量越大。
strides: 步长,shape为1, stride_h, stride_w, 1,通常stride_h和stride_w相等,表示卷积核延纵横方向上每次前进的步数。上gif图stride为1。
padding:卷积计算时数据不对齐时填充方式,VALID:丢弃多余;SAME:两端补0,让多余部分可被计算。
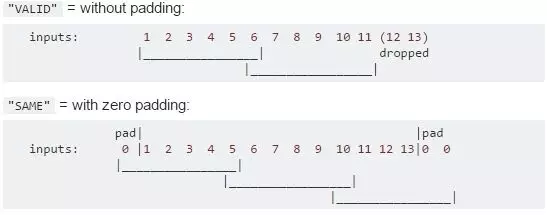
output:输出,shape为batch, out_height, out_width, out_channels

激活函数,与卷积搭配使用。激活函数不是真的要去激活什么,在神经网络中,激活函数的作用是能够给神经网络加入一些非线性因素,使得神经网络可以更好地解决较为复杂的问题。

tf.nn.relu 即是激活函数,对卷积输出作非线性处理,其函数如下:
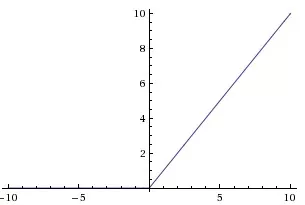
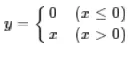
其它还有如 sigmoid:
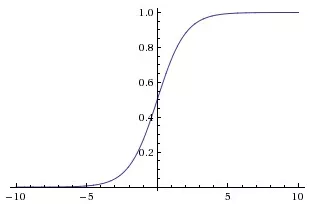
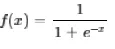
tanh:
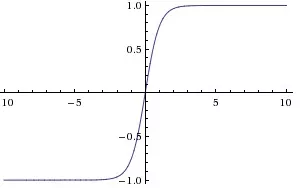
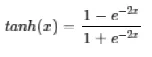
Pool 池化,有最大池化和平均值池化,计算与卷积计算类似,但无卷积核,求核所覆盖范围的最大值或平均值,输入 channel 对应输出 channel,没有多层累加情况。输入与输出 channel 数相同,输出 height、width 取决于 strides。
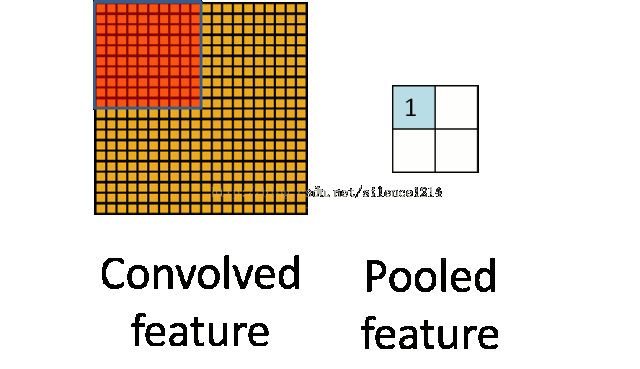
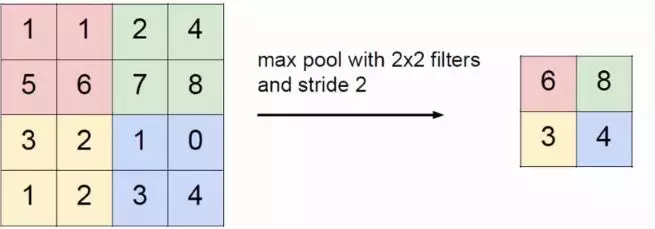

Dropout,随机删除一些数据,让网络在这些删除的数据上也能训练出准确的结果,让网络有更强的适应性,减少过拟合。

BN(batch normalize),批规范化。Inference中未标出,demo中未使用,但也是网络中很常用的一层。BN常作用在非线性映射前,即对Conv结果做规范化。一般的顺序是 卷积-> BN -> 激活函数。
BN 好处:提升训练速度,加快 loss 收敛,增加网络适应性,一定程序的解决反向传播过程中的梯度消失和爆炸问题。详细请戳。
FC(Full Connection)全连接,核心是矩阵相乘

,softmax 性线回归就是一个 FC。在 CNN 中全连接常出现在最后几层,用于对前面设计的特征做加权和。Tensorflow 提供了相应函数 tf.layers.dense。
日志,下图打印了模型中需要训练的参数的shape 和 各层输出数据的shape(batch_size=1时),附件【tool.py】中有相关代码。目的是方便观自己搭的网络结构是否符合预期。 数据由1x784] -reshape-> [1x28x28x1 -conv-> [1x28x28x32] -pool-> [1x14x14x32] -conv-> [1x14x14x64] -pool-> [1x7x7x64] -fc-> [1x1024] -fc-> [1x10](每类数字的概率)
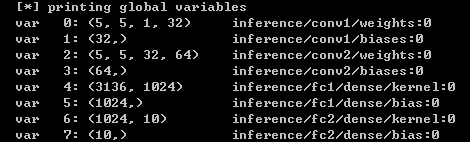
训练效果,详细代码参考附件【cnn.py】
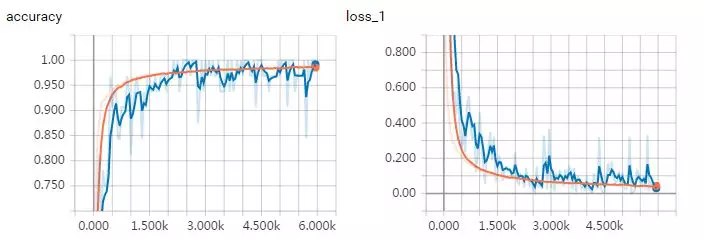
一个网上的可视化手写识别DEMO,http://scs.ryerson.ca/~aharley/vis/conv/flat.html
CNN家族经典网络,如LeNet,AlexNet,VGG-Net,GoogLeNet,ResNet、U-Net、FPN。它们也都是由基本网络层元素(上述介绍)堆叠而成,像搭积木一样。
VGG,如下图,非常有名的特征提取和分类网络。由多层卷积池化层组成,最后用 FC 做特征融合实现分类,很多网络基于其前几层卷积池化层做特征提取,再发展自己的业务。
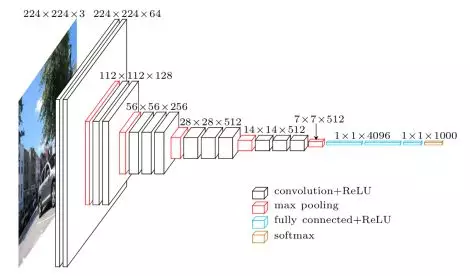
5 tool 工具类
【tool.py】是一个自己基于 tensorflow 二次封装的工具类,位于附件中。好处是以后编程更方便,代码结构更好看。网上也有现成的开源库,如 TensorLayer、Keras、Tflearn,自己封装的目的是更好的理解 tensorflow API,自己造可控性也更强一些,如果控制是参数是否被训练、log 打印。
下图是 MNIST CNN 网络的 Inference 推理代码:
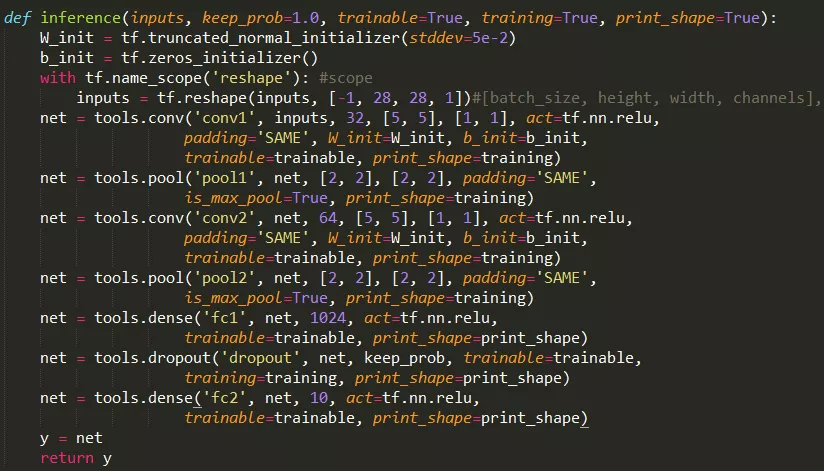
6 CPU & GPU & multi GPU
CPU, Tensorflow默认所有cpu都是/cpu:0,默认占所有cpu,可以通过代码指定占用数。

GPU,Tensorflow默认占用/gpu:0, 可通过指定device来确定代码运行在哪个gpu。下面
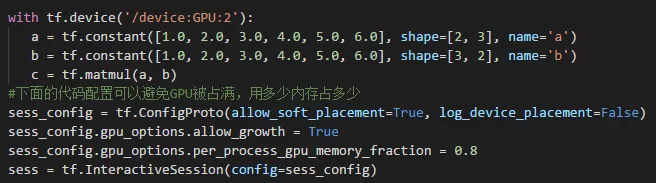
多块 GPU 时,可以通过在终端运行下面指令来设置 CUDA 可见 GPU 块来控制程序使用哪些 GPU。

多GPU使用,在Tensorflow中多GPU编程比较尴尬,资料较好,代码写起比较复杂,这一点不如Caffe。
在 Tensorflow 中你需要自己写代码控制多 GPU 的 loss 和 gradient 的合并,这里有个官方例子请戳。自己也写过多 GPU 的工程,附件代码【tmp-main-gpus-不可用.py】可做参考,但此处不可用,来自它工程。
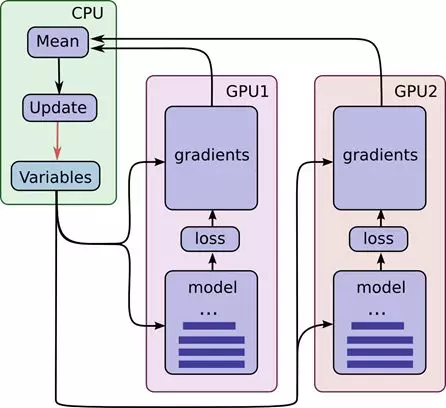
本文转载自公众号云加社区(ID:QcloudCommunity)。
原文链接:
https://mp.weixin.qq.com/s/by7mj8o4nn_I1X2GklLGsA
评论