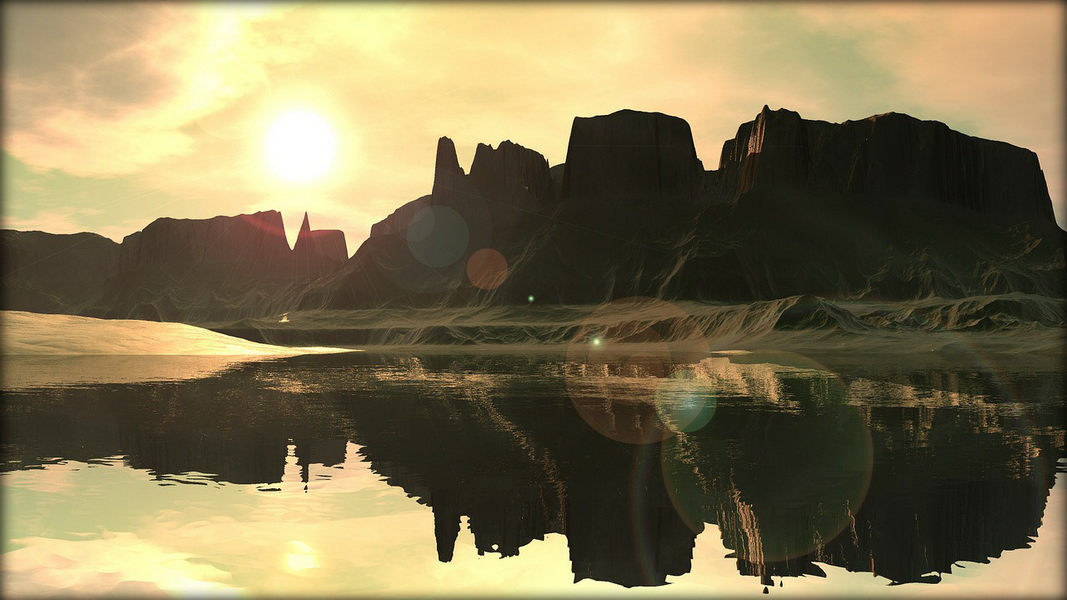
在 ArchSummit 北京 2019 大会上,陈海春讲师做了《AI / ML 算法的公平性,偏差和对社会/经济的影响》主题演讲,主要内容如下。
演讲简介:
Fairness, bias, and social/economical impact of AI/ML algorithms
“With great power comes great responsibility”, as AI/ML especially deep learning continues to advance in research and expand to commercial applications, AI/ML algorithms are making big social economical impact to people’s lives, from deciding what health insurance policy a person can get, to whether a bank decides to issue a loan to a borrower, or what content a person can see on a web site. With even a slight bias, the algorithms can amplify unfairness or even injustice. So how do we unleash the power of AI/ML to improve people’s lives with fairness and justice, while not tying the hands of the algorithm developers? In this talk, I will talk about how bias creeps into your ML models, both consciously and unconsciously, both from data and from the code, how to address them with novel debias techniques and blackbox model interpretation components, and how to design fairness principles into the architecture of your ML platform, all by using real world examples, cutting edge research results, and practical techniques in algorithm and architecture design. At the end of the talk, you should have a higher level of awareness of bias in AI/ML algorithms, recognize the value of fairness instead of viewing it as an inconvenience, have a mindset of how to address them in your design of ML platform and solutions.
内容大纲:
Overview of fairness and bias in AI/ML
Unconscious bias in data
Unconscious bias in algorithms
Well-known trust busters
Current state of the art: research and industry
Case study: B2B AI/ML solutions for digital experience optimization
Identification of protected groups
Generic measurement of fairness
Innovation to correct bias while minimizing accuracy loss
Innovation to interpret black-box model results
Opportunities and challenges in generalizing fairness practices in AI/ML platforms
参考译文:
演讲简介:
俗话说“权力越大责任越大”。随着 AI / ML(尤其是深度学习)在研究中不断发展并扩展到商业应用,AI / ML 算法对人们的生活日渐产生巨大的社会经济影响,例如保险公司卖给消费者什么样的健康保险,银行是否决定向借款人发放贷款,甚至在网站决定访问者看到什么内容都是由算法决定。即使有轻微的偏差,这些算法都会加剧社会的不公平甚至不公正。那么,如何在不束缚算法开发人员创新的前提下,发挥 AI / ML 的力量,以公平和正义的方式来改善人们的生活呢?
在本次演讲中,我将讨论数据和算法如何有意识或无意识地将偏差渗入到 ML 模型中,如何使用新颖的 Debias 技术和黑盒式模型解释组件来解决这个问题,以及如何将公平原则融入到 ML 平台的架构设计中。所有这些都通过真实案例,前沿研究结果以及算法和架构设计中的实用技术来讲述,以帮助大家对 AI / ML 算法中的偏差有更高的认识,认识到公平的价值,而不是将其视为负担,同时也了解如何在 ML 平台设计中处理这些问题。
内容大纲:
AI / ML 中的公平性和偏差概述
数据中的无意识偏差
算法中的无意识偏差
著名的信任破坏案例
最新的研究和工业技术案例
案例研究:用于数字体验优化的 B2B AI / ML 解决方案
确认受保护群体
公平性的衡量方法
最小精度损失的去偏差创新方法
黑箱式模型解释创新方法
在 AI / ML 平台中推广公平实践的机会和挑战
讲师介绍:
陈海春
Netflix Manager, Content Knowledge, Data Science and Engineering
I am managing Content Knowledge Graph team at Netflix where we apply deep learning techniques in NLP and CV to curate the best knowledge about entities in the entertainment world, which in turn is used in content/talent discovery and acquisition. We leverage state-of-the-art models but also develop our own technology whenever necessary. Prior to Netflix, I led data science teams as a group manager at Adobe Inc. where my teams provided AI/ML solutions to enterprises such as Nike and Disney. Prior to Adobe I worked as a senior machine learning engineer at Google where I applied AI/ML techniques to combat abuse and anomaly problems for highly impactful products such a AdWords and Google Play. Prior to Google, I worked as Software Architect at Synopsys Inc. where I applied algorithms and system design to solve simulation problems for semiconductor design and manufacturing.
参考译文:我在 Netflix 带领内容知识图谱团队,结合 NLP 和 CV 中的深度学习技术,来收集有关娱乐界个体的最准确知识,然后利用这些知识帮助我们发现和招募优秀的娱乐内容和人才。我们尽可能采用当前最先进的模型,如有需要也会开发自己的技术。在加入 Netflix 之前,我曾在 Adobe Inc. 领导数据科学团队,为耐克和迪斯尼等企业提供 AI / ML 解决方案。在此之前,我在 Google 担任高级机器学习工程师,使用 AI / ML 技术来解决 AdWords 和 Google Play 等产品中的滥用和异常行为侦测。在 Google 之前,我曾在 Synopsys Inc. 担任软件架构师,应用算法和系统设计来解决半导体设计和制造中的仿真问题。
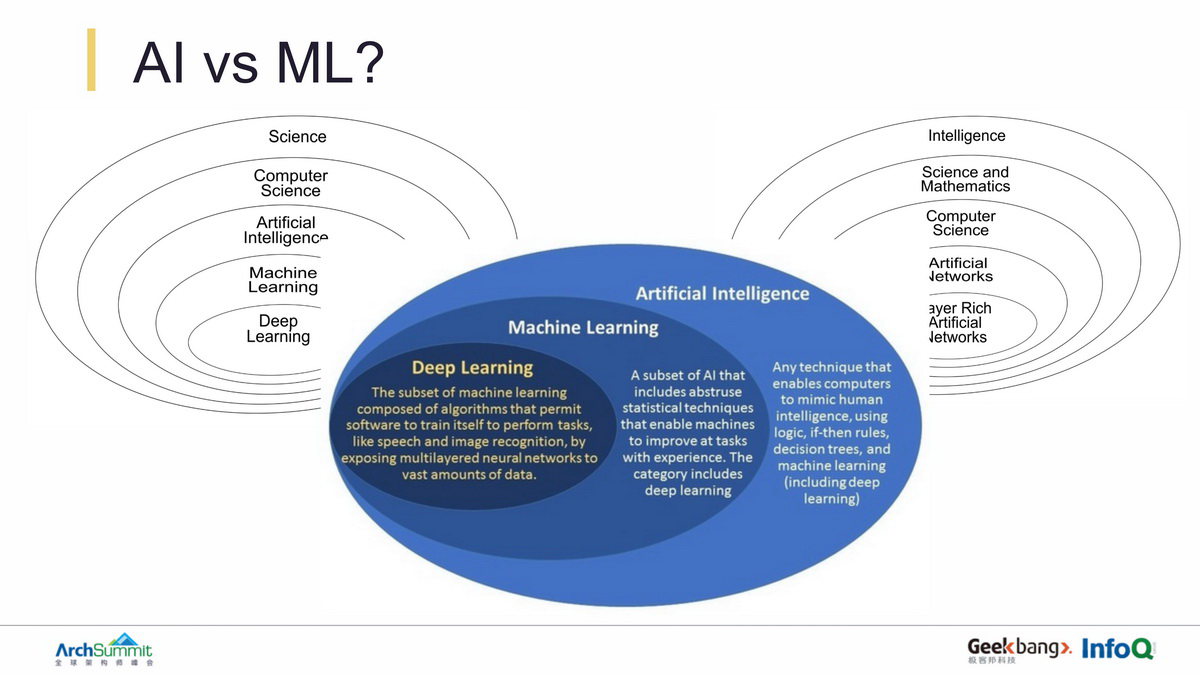
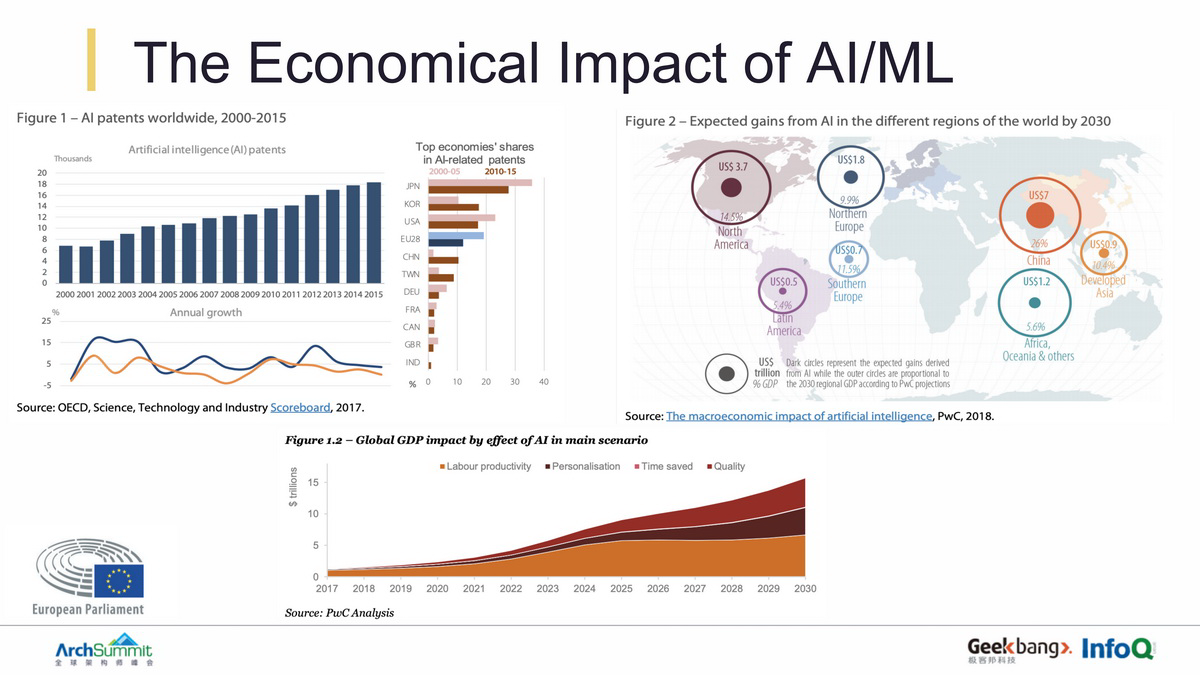
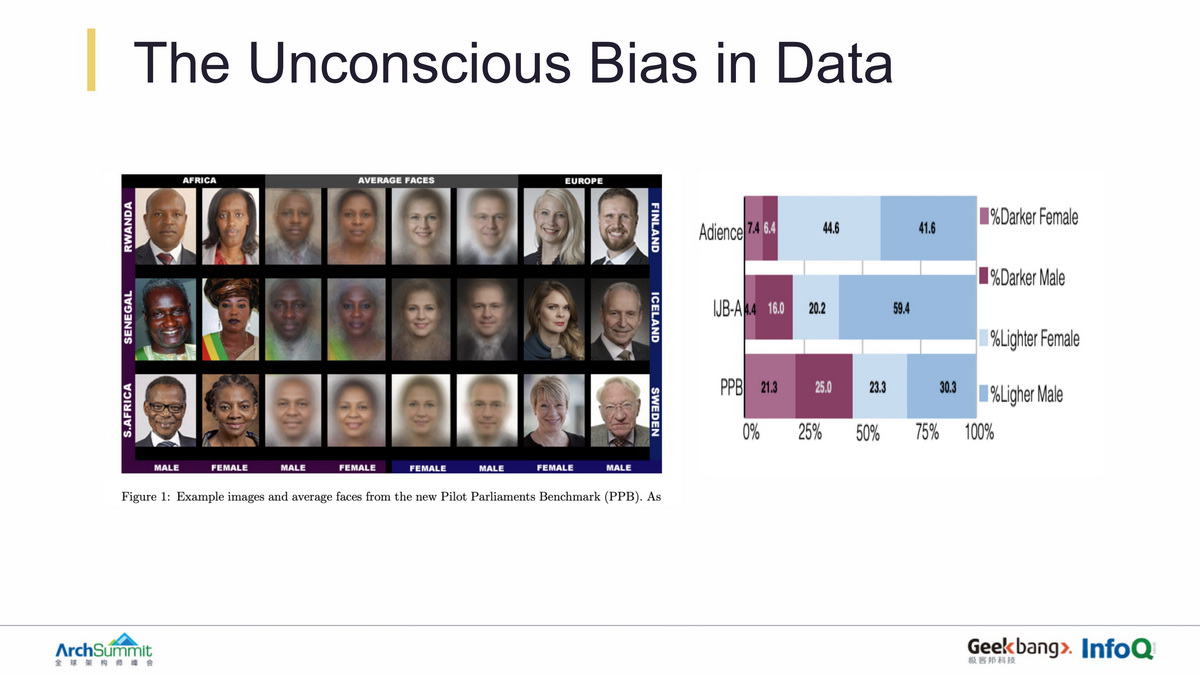
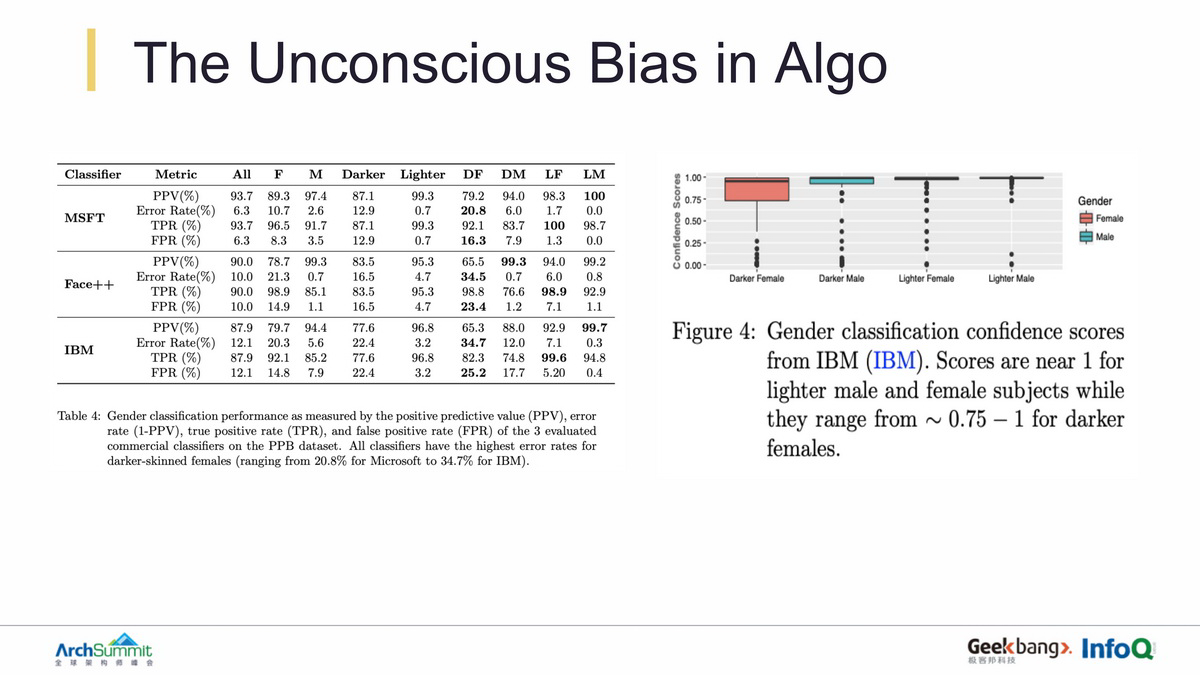
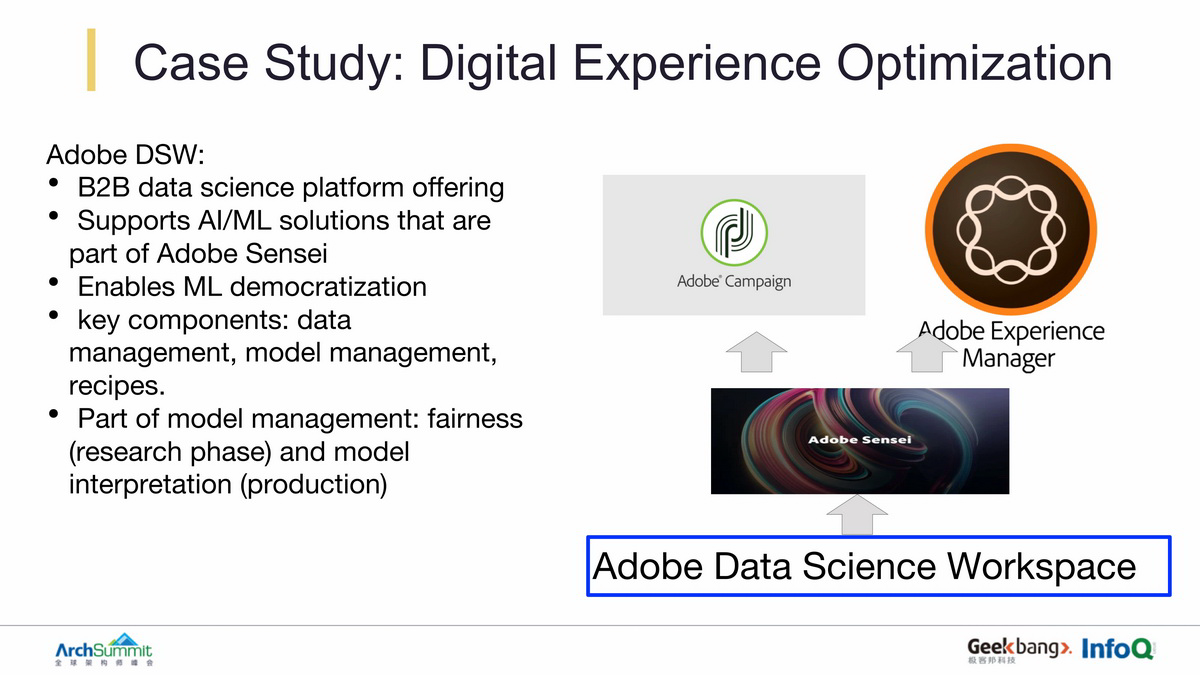
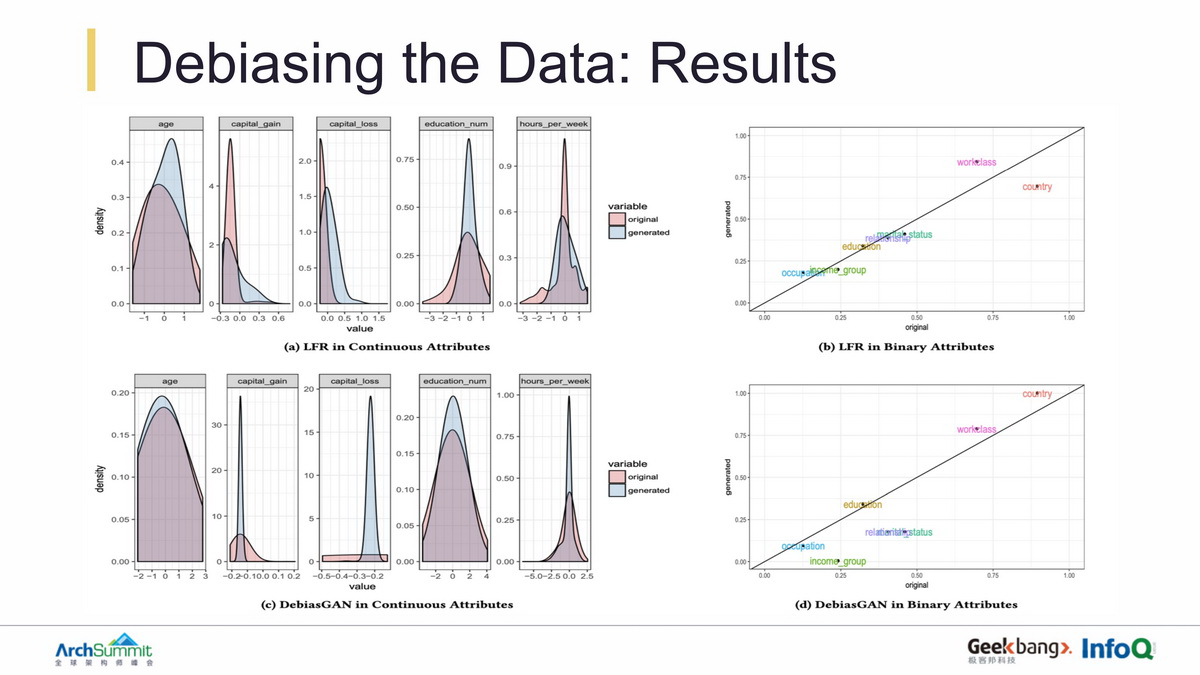
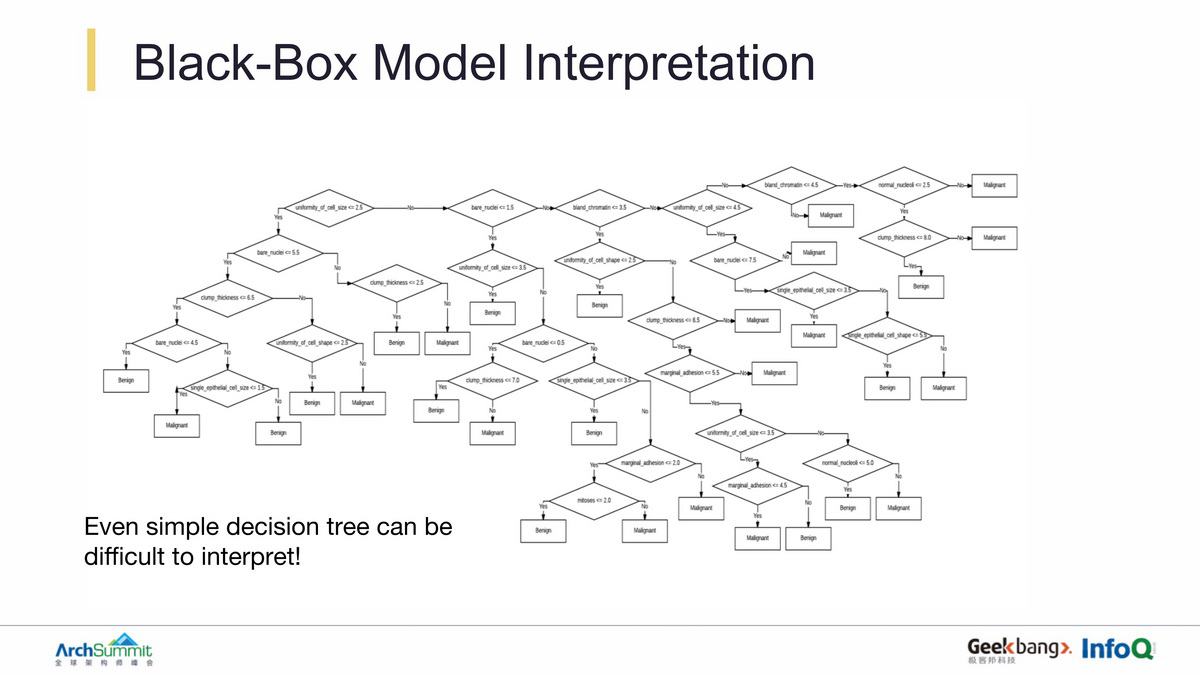
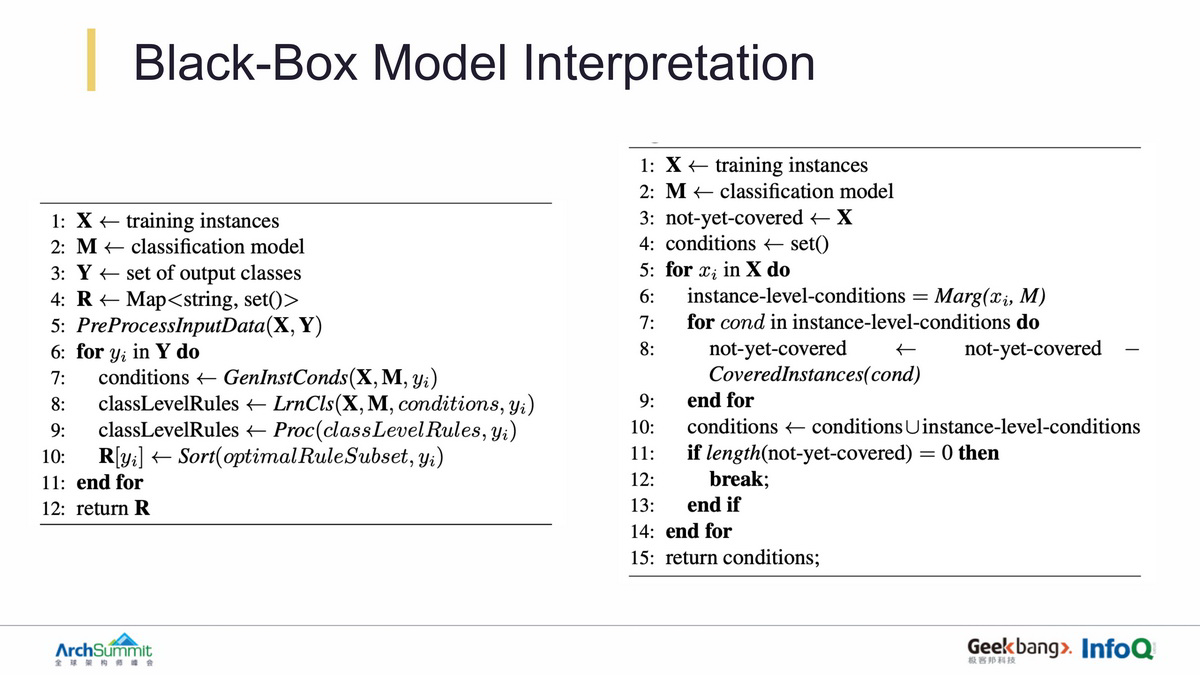
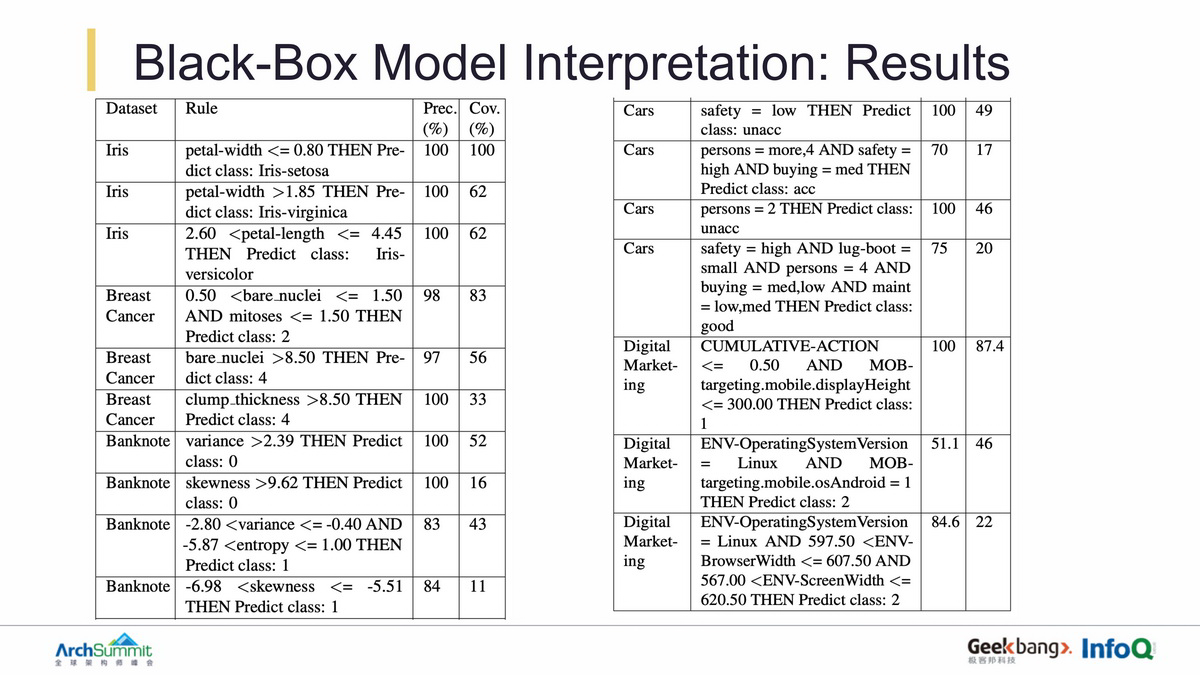
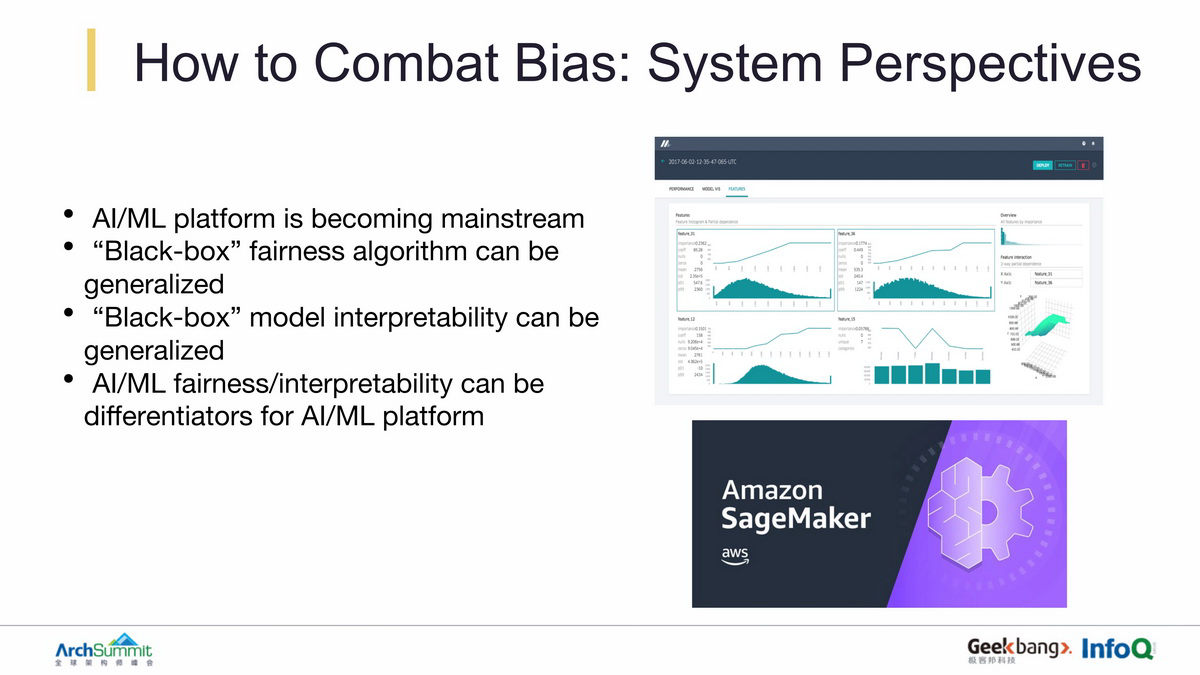
完整演讲 PPT 下载链接:
https://archsummit.infoq.cn/2019/beijing/schedule
评论