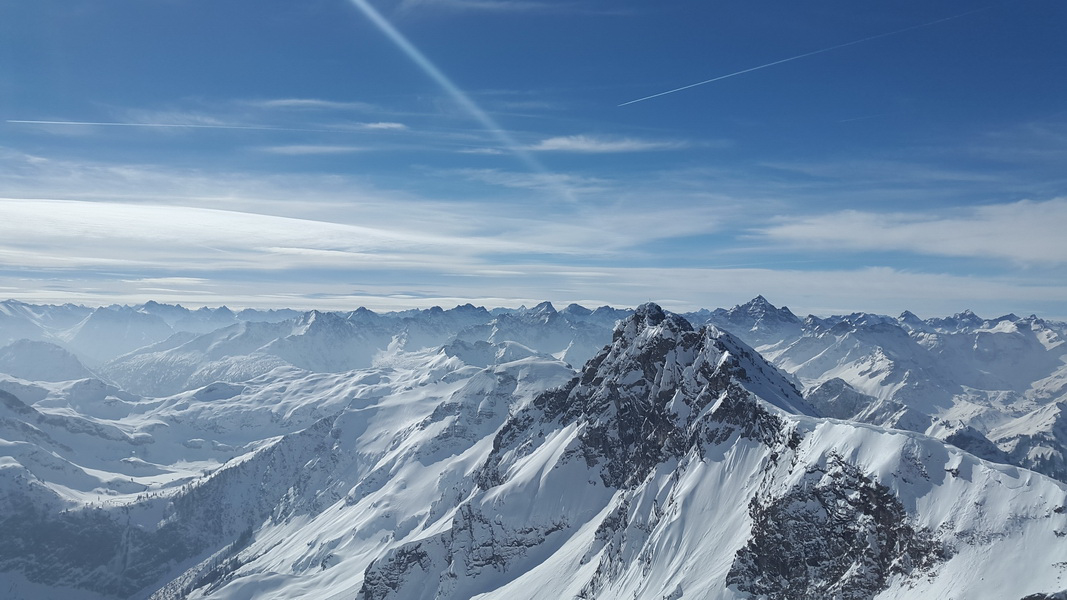
在ArchSummit深圳2019大会上,蔡東邦 (DB Tsai)讲师做了《如何弥合 Spark Datasets 和 DataFrames 之间的性能差距?》主题演讲,主要内容如下。
演讲简介:
Bridging the gap between Spark Datasets and DataFrames
Apple leverages Apache Spark for processing large datasets to power key components of Apple’s production services. The majority of users rely on Spark SQL to benefit from state-of-the-art optimizations in Catalyst and Tungsten. As there are multiple APIs to interact with Spark SQL, users have to make a wise decision which one to pick. While DataFrames and SQL are widely used, they lack type safety so that the analysis errors will not be detected during the compile time such as invalid column names or types. Also, the ability to apply the same functional constructions as on RDDs is missing in DataFrames. Datasets expose a type-safe API and support for user-defined closures at the cost of performance.
译文参考:
Apple 利用 Apache Spark 处理大型数据集,为 Apple 生产服务的关键组件提供动力。 大多数用户依靠 Spark SQL 从 Catalyst 和 Tungsten 中最先进的优化中受益。由于有多个 API 与 Spark SQL 交互,因此用户必须明智地决定最终选择哪一个。虽然 DataFrame 和 SQL 被广泛使用,但它们缺乏类型安全性,因此在编译期间无法检测到分析错误,例如无效的列名称或类型。此外,在 RDDs 上应用相同功能结构的功能却不能在 DataFrames 中使用。Datasets 以牺牲性能为代价公开了类型安全的 API,并支持用户定义的闭包。
This talk will explain cases when Spark SQL cannot optimize typed Datasets as much as it can optimize DataFrames. We will also present an effort to use bytecode analysis to convert user-defined closures into native Catalyst expressions. This helps Spark to avoid the expensive conversion between the internal format and JVM objects as well as to leverage more Catalyst optimizations. A consequence, we can bridge the gap in performance between Datasets and DataFrames, so that users do not have to sacrifice the benefits of Datasets for performance reasons.
本演讲将重点介绍 Spark SQL 无法优化类型化 Datasets 的情况,因为它可以优化 DataFrame。我们还将努力使用字节码分析将用户定义的闭包转换为本机 Catalyst 表达式。这有助于 Spark 避免在内部格式和 JVM 对象之间的昂贵转换,以及利用更多的 Catalyst 优化。因此,我们可以弥合 Datasets 和 DataFrames 之间的性能差距,这样用户就不会因性能原因而牺牲 Datasets 的优势。
Outlines of the speech:
Apache Spark
Dataframe vs Dataset
Bytecode Analysis
JVM GC
Key points:
The audiences will learn how Apache Spark works internally; particularly on how catalyst optimizer optimizes the execution plans.
Then I will go through the current problems of Spark’s Dataset implementation which expose a type-safe API and support for user-defined closures at the cost of performance.
Finally, I’ll talk about how we do bytecode analysis to understand the JVM bytecode, and then convert them into SQL expressions for further optimization. In some of our Spark jobs, we see 40x performance gain resulting less required resource and faster deliver time.
帮助听众了解 Apache Spark 内部的工作流程,尤其是 Catalyst 优化器如何优化执行计划。
了解 Spark Dataset 当前在应用方面的问题,这些问题公开了类型安全的 API,并以性能为代价支持用户定义的闭包。
最后,我将分享我们是如何实现字节码分析的案例,帮助理解 JVM 字节码,然后将它们转换为 SQL 表达式以进一步优化。在我们所做的一些 Spark 工作中,我们看到了 40 倍的性能提升,从而减少了所需的资源,交付时间也更快。
讲师介绍:
蔡東邦 (DB Tsai)
Apple Staff Software Engineer & Apache Spark PMC
DB Tsai is an Apache Spark PMC / Committer and an open source and staff software engineer at Apple Siri. He implemented several algorithms including linear models with Elastici-Net (L1/L2) regularization using LBFGS/OWL-QN optimizers in Apache Spark. Prior to joining Apple, DB worked on Personalized Recommendation ML Algorithms at Netflix. DB was a Ph.D. candidate in Applied Physics at Stanford University. He holds a Master’s degree in Electrical Engineering from Stanford.
译文参考:
蔡東邦老师是 Apache Spark PMC / Committer,同时也是 Apple Siri 的主任工程师。他将多个算法应用到了 Apache Spark 当中,包括使用了 LBFGS / OWL-QN 优化器 的 Elastici-Net(L1 / L2)正则化的线性模型。在加入 Apple Siri 之前,蔡老师在 Netflix 从事个性化推荐机器学习算法的研究工作。目前是斯坦福大学应用物理专业的博士候选人,也获得了斯坦福大学电气工程硕士学位。
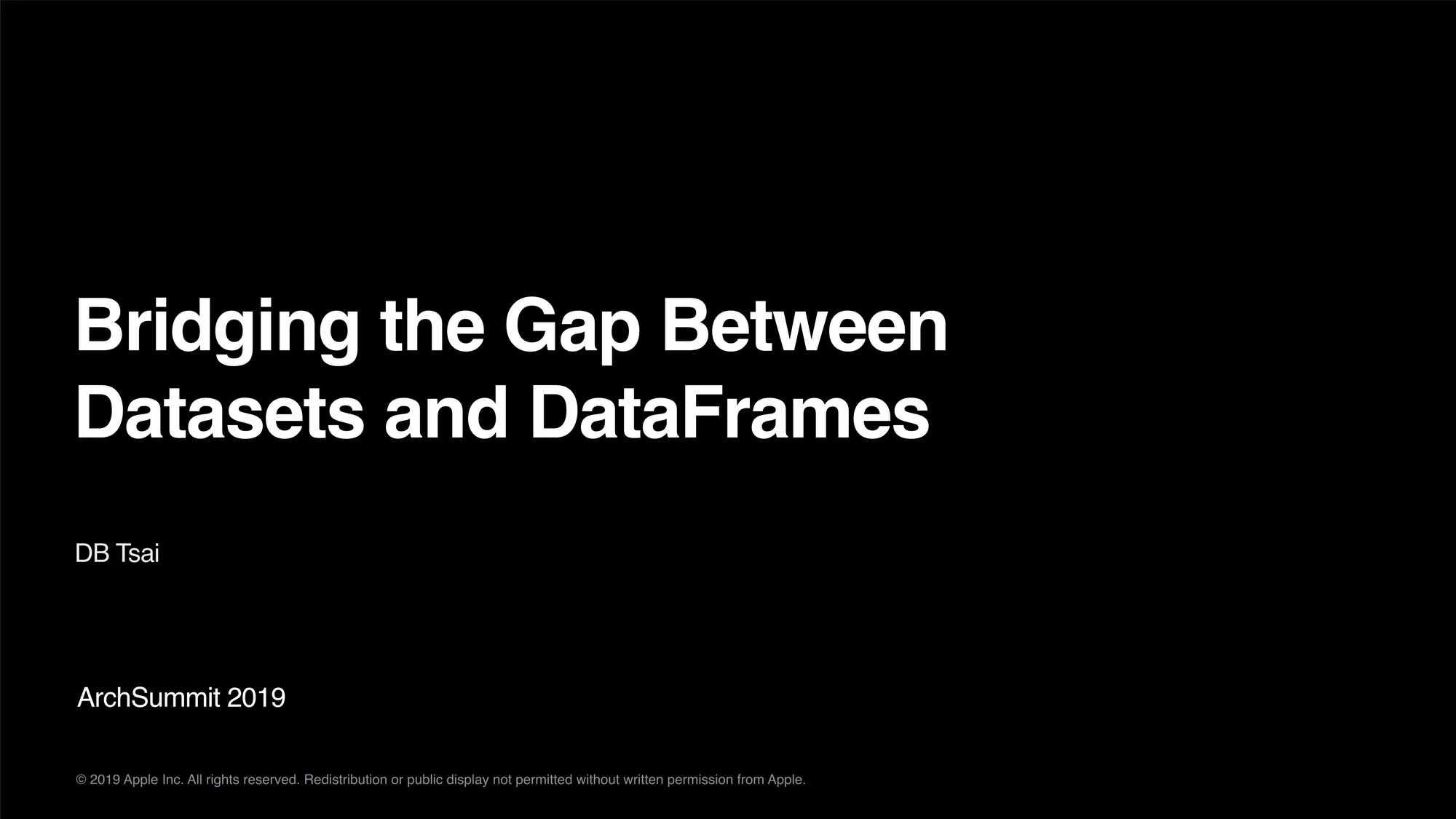
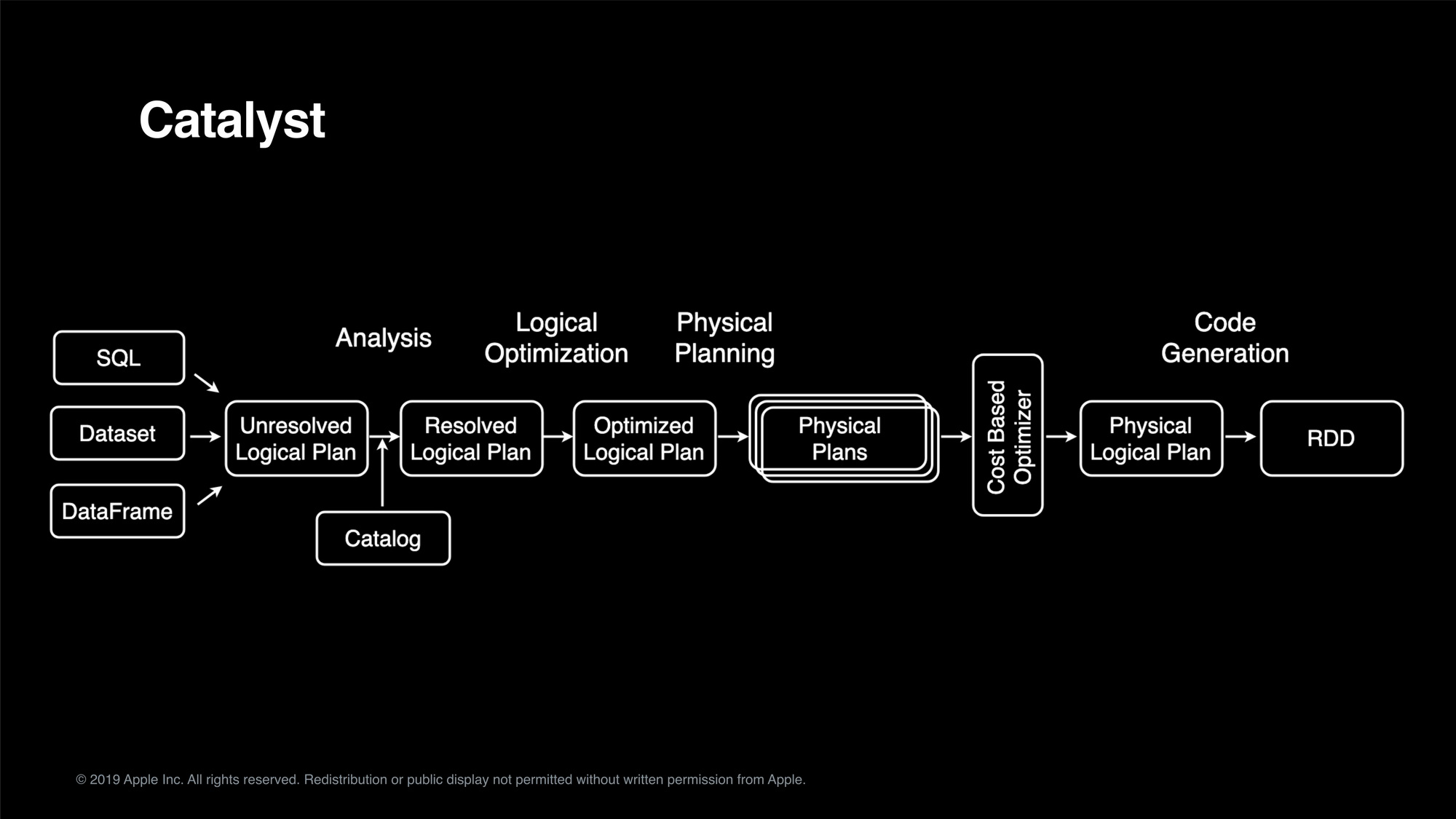
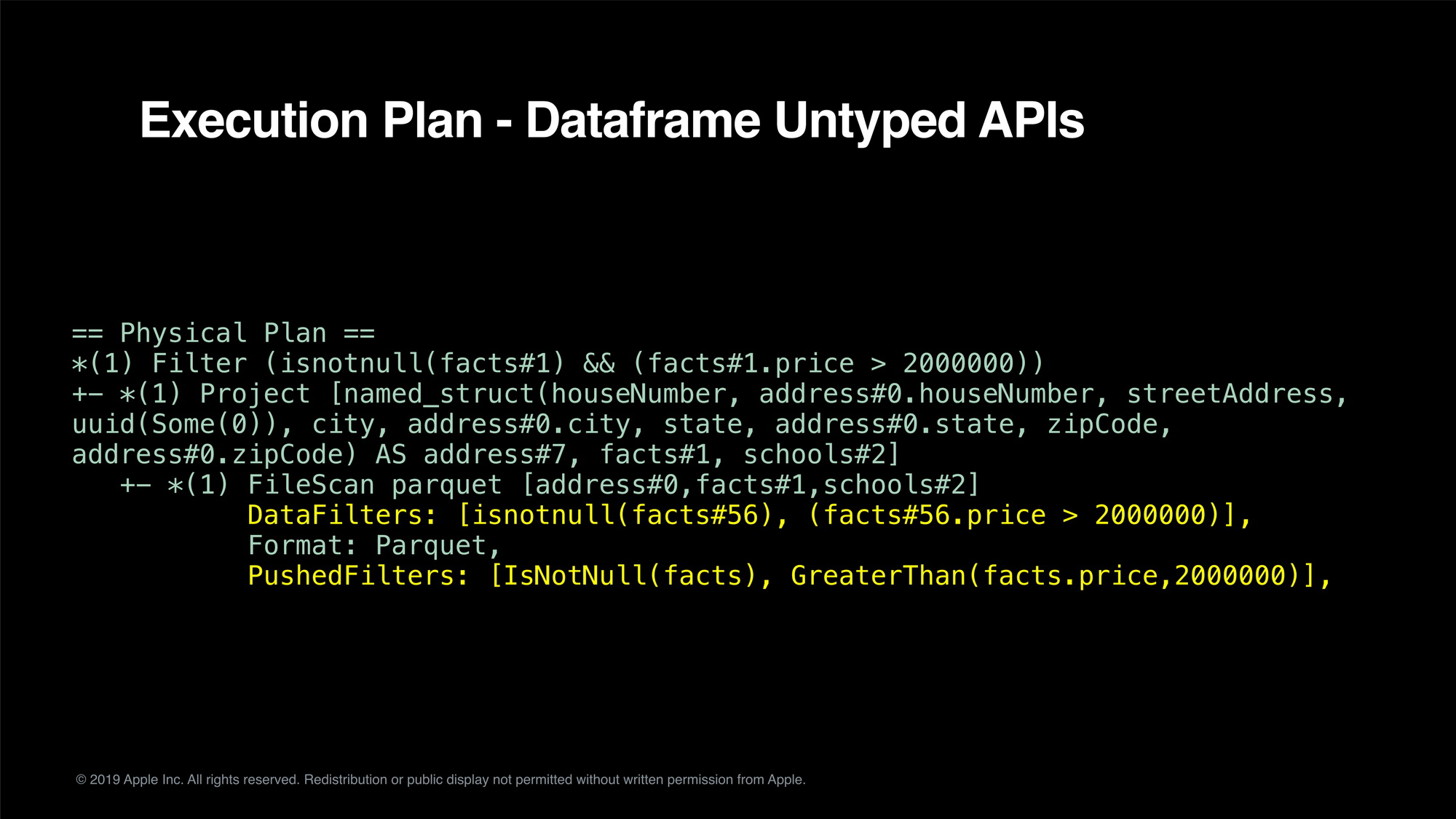
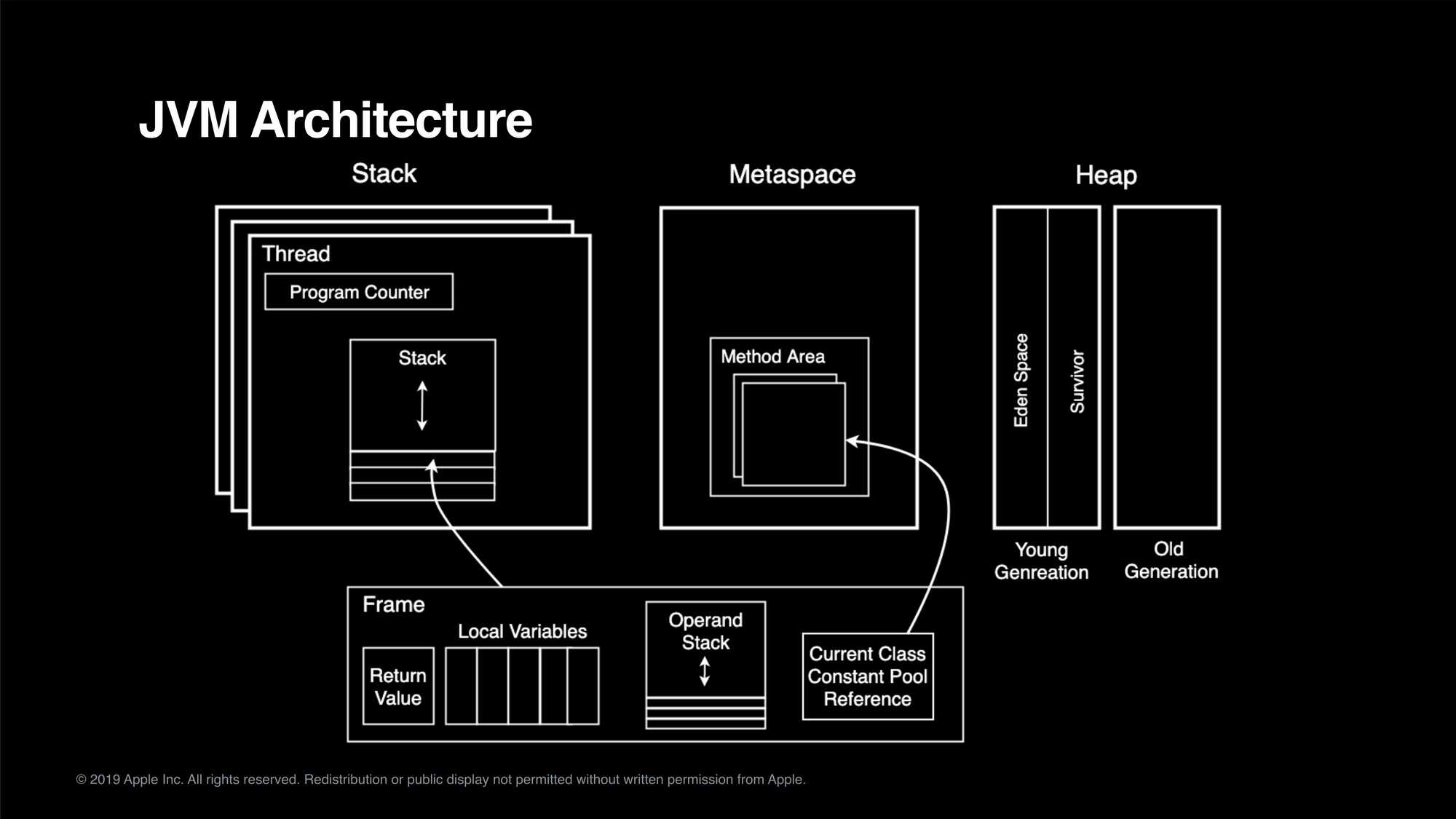
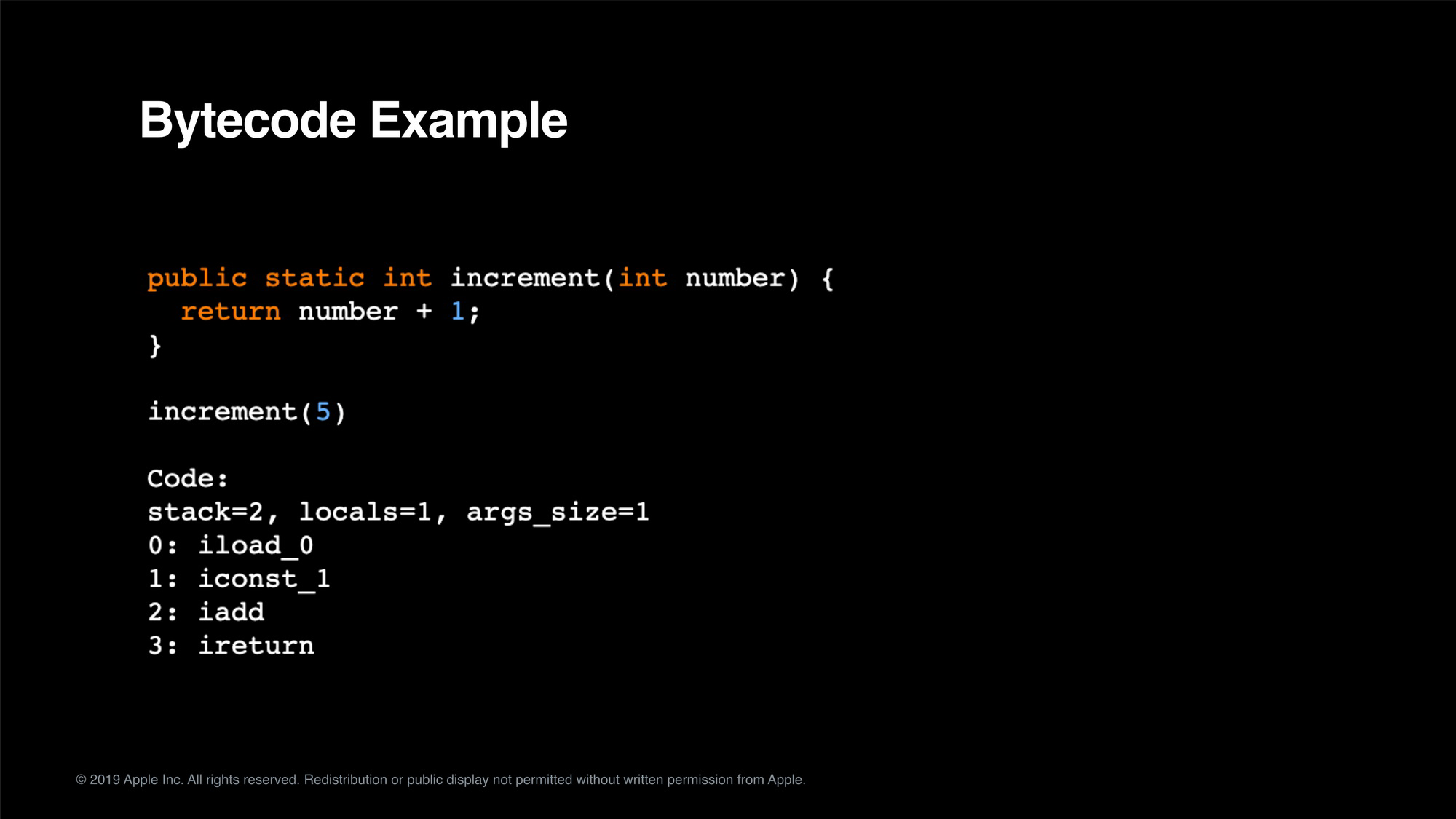
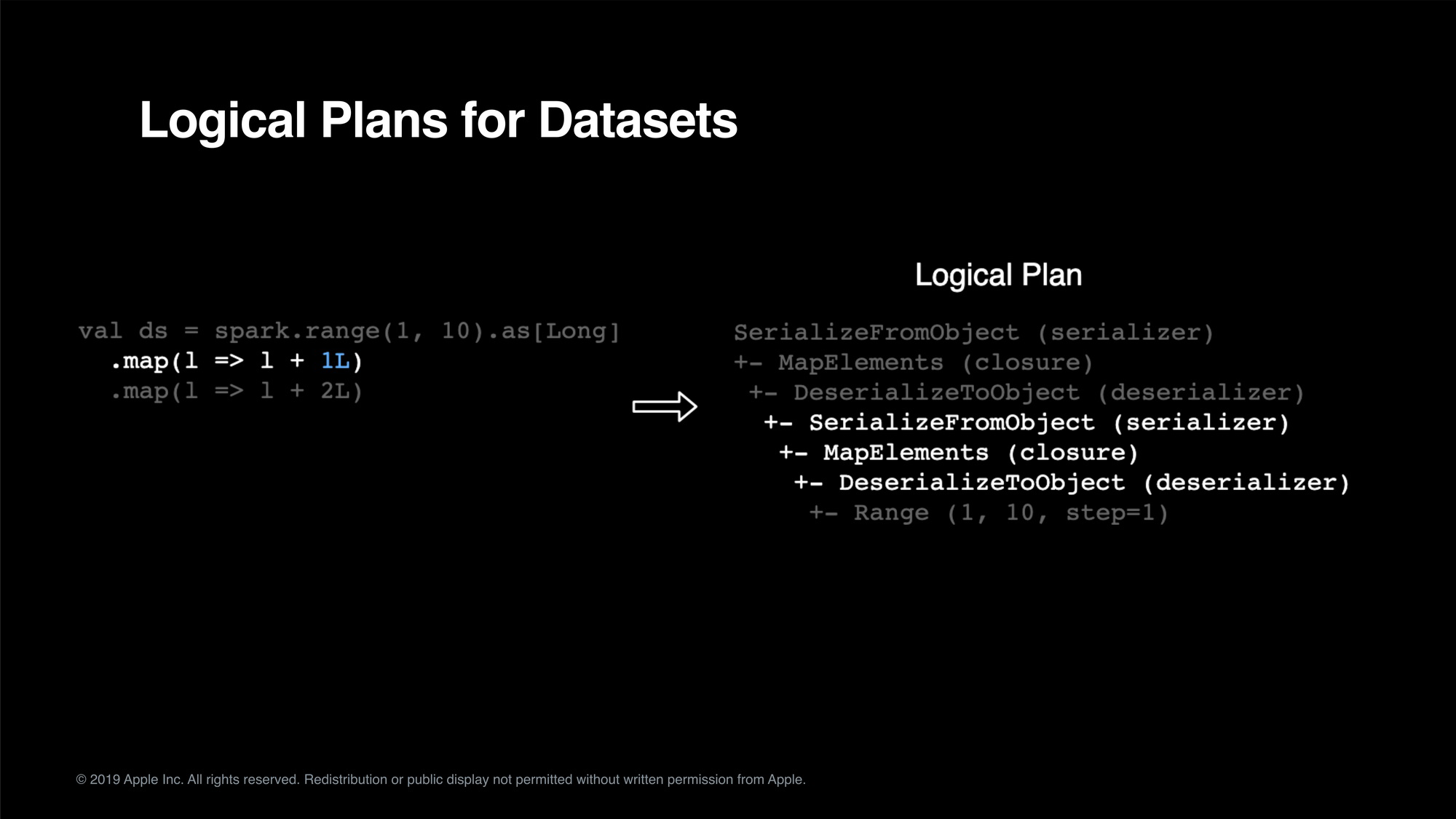
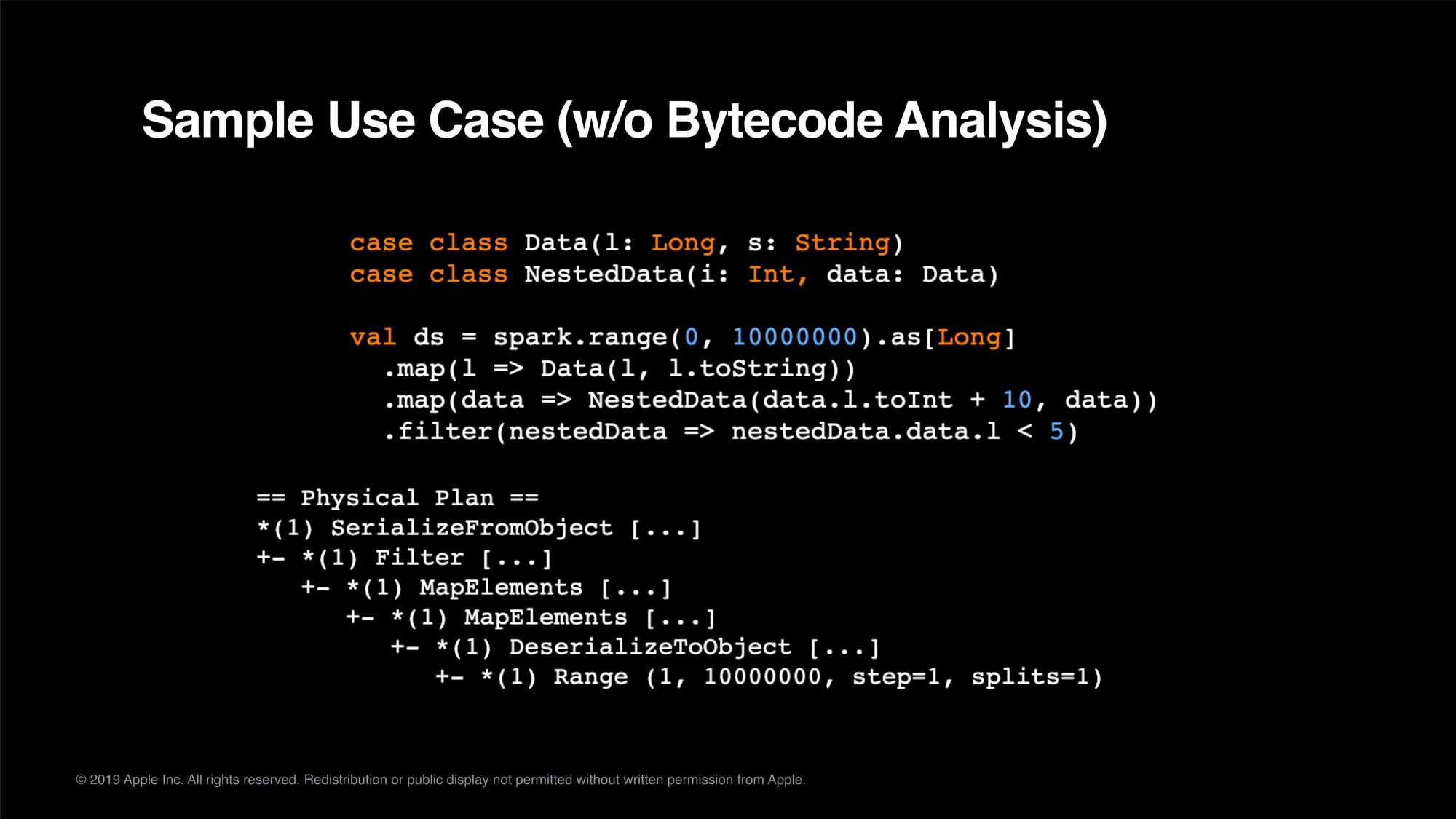
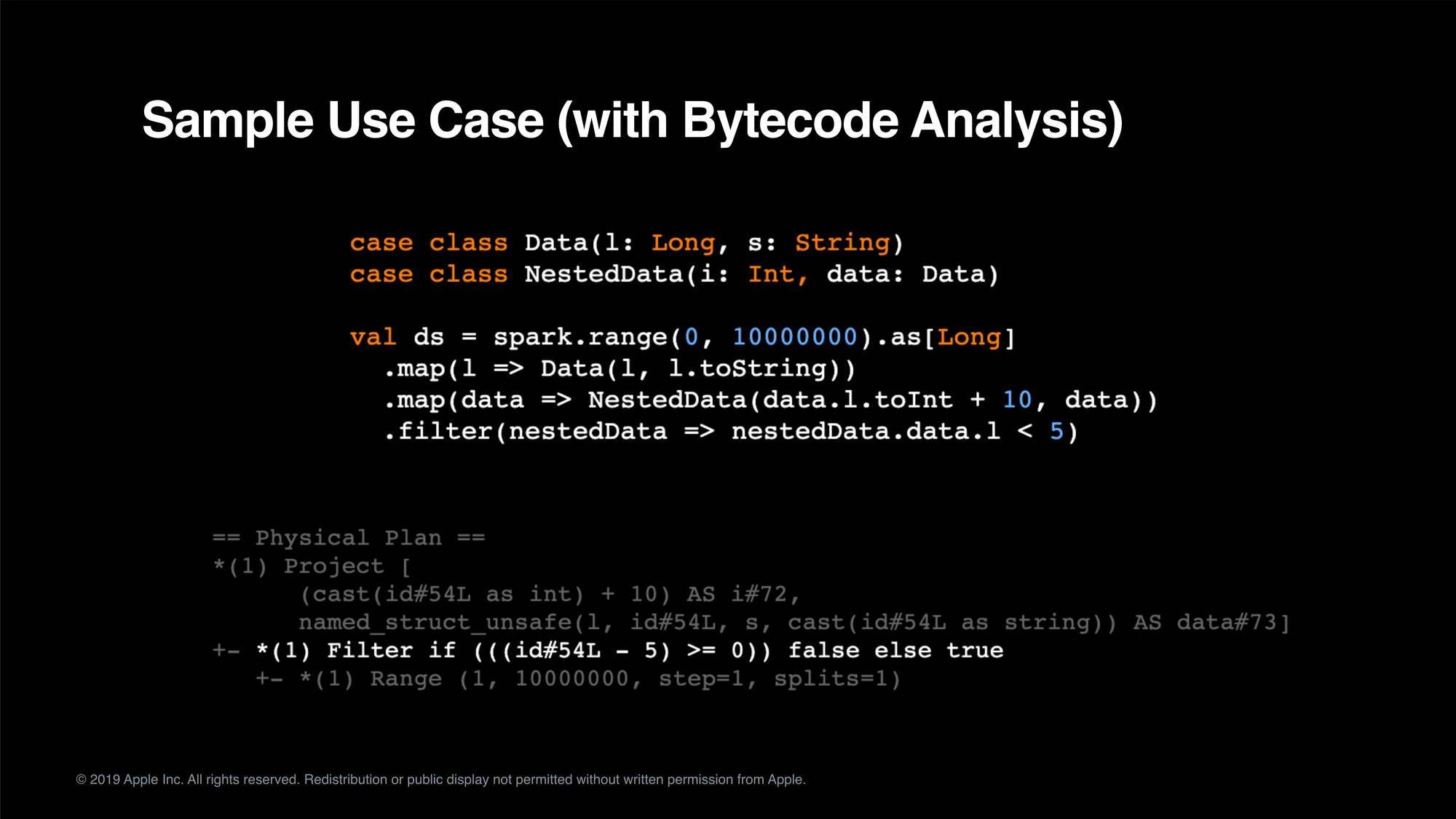
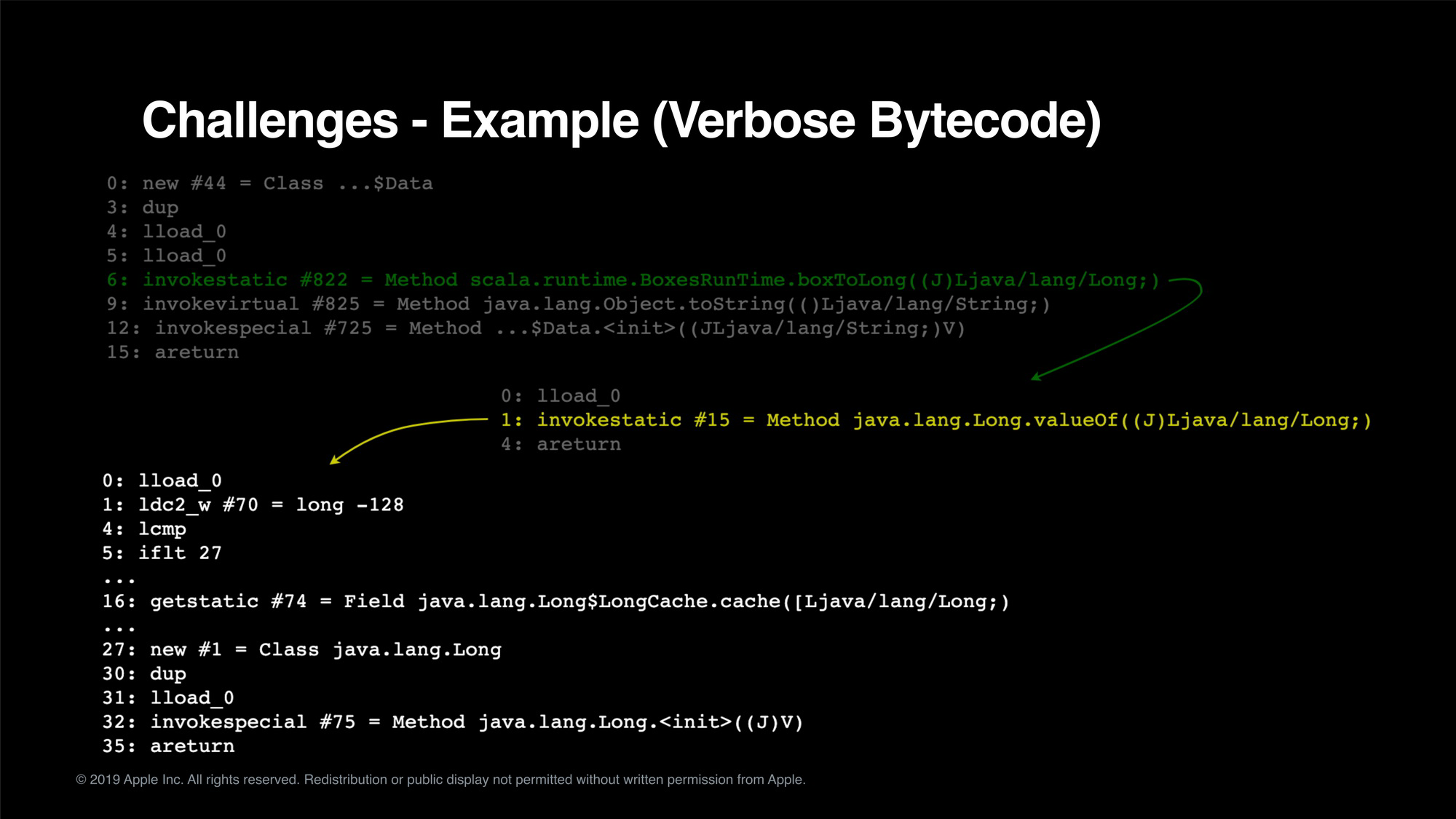
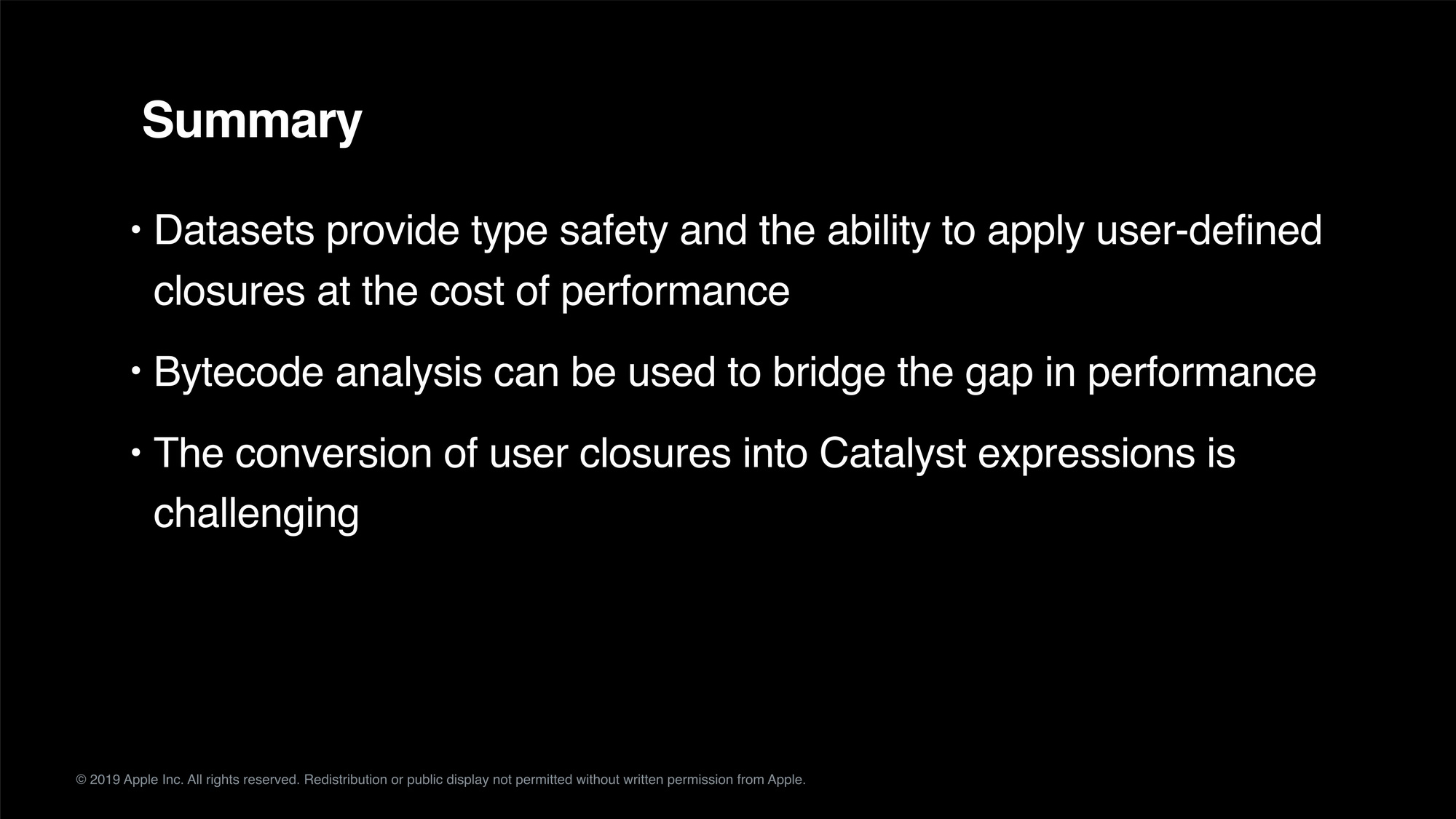
完整演讲 PPT 下载链接:
https://archsummit.infoq.cn/2019/shenzhen/schedule
评论